On-Demand Videos
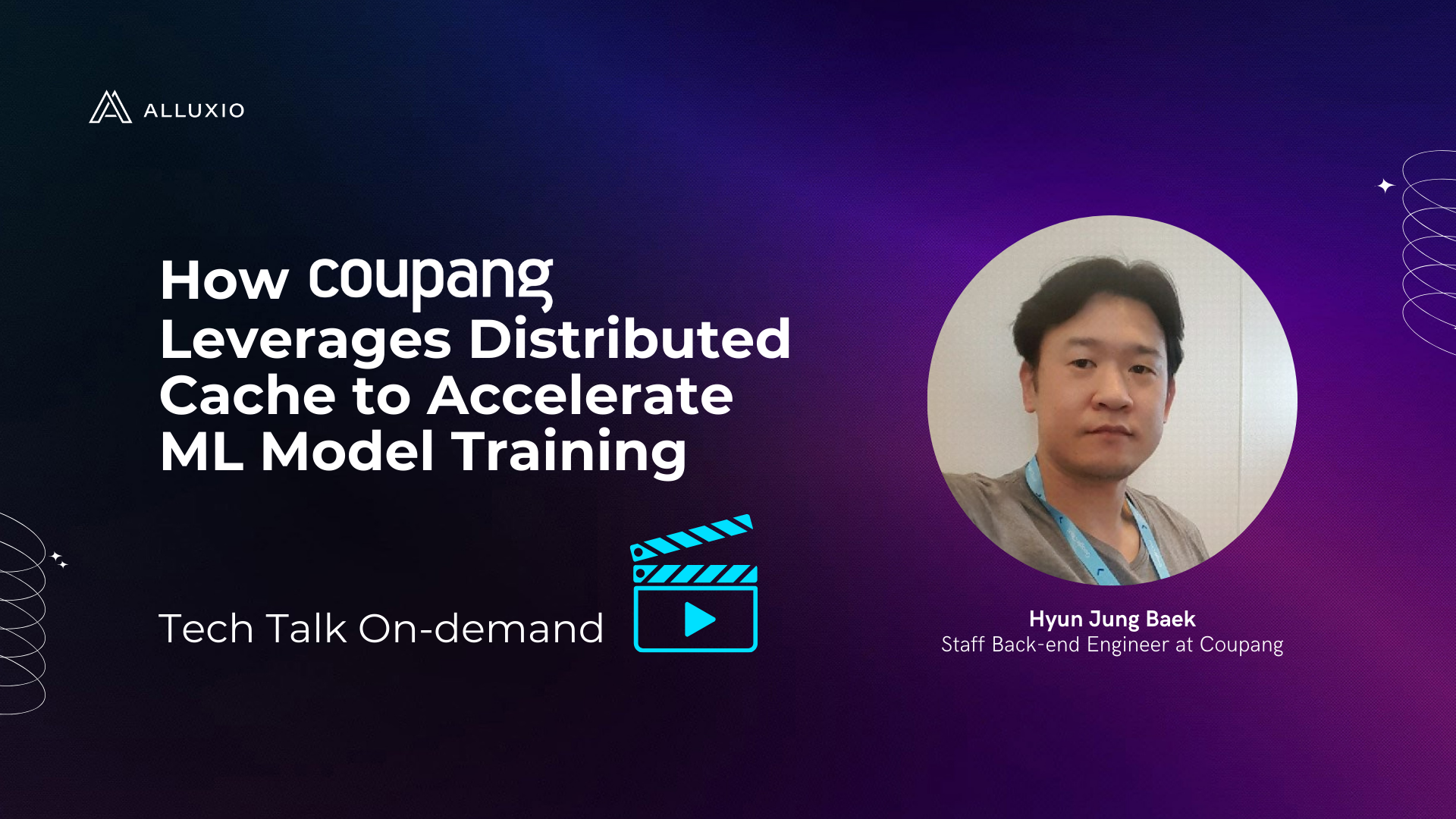
Coupang is a leading e-commerce company in South Korea, with over 50,000 employees and $20+ billion in annual revenue. Coupang's AI platform team builds and manages a large-scale AI platform in AWS for machine learning engineers to train models that enhance and customize product search results and product recommendations for its 100+ million customers.
As the search and recommendation models evolve, optimizing the underlying infrastructure for AI/ML workloads is essential for the e-commerce business. Coupang's platform team actively sought to improve their model training pipeline to boost machine learning engineers' productivity, publish models to production faster, and reduce operational costs.
Coupang focused on addressing several key areas:
- Shortening data preparation and model training time
- Improving GPU utilization in training clusters in different regions
- Reducing S3 API and egress costs incurred from copying large training datasets across regions
- Simplifying the operational complexity of storage system management
In this tech talk, Hyun Jung Baek, Staff Backend Engineer at Coupang, will share best practices for leveraging distributed caching to power search and recommendation model training infrastructure.
Hyun will discuss:
- How Coupang builds a world-class large-scale AI platform for machine learning engineers to deliver better search and recommendation models
- How adding distributed caching to their multi-region AI infrastructure improves GPU utilization, accelerates end-to-end training time, and significantly reduces cross-region data transfer costs.
- How to simplify platform operations and to easily deploy the same architecture to new GPU clusters.
About the Speaker
Hyun Jung Baek is a Staff Backend Engineer at Coupang.
Deepseek’s recent announcement of the Fire-flyer File System (3FS) has sparked excitement across the AI infra community, promising a breakthrough in how machine learning models access and process data.
In this webinar, an expert in distributed systems and AI infrastructure will take you inside Deepseek 3FS, the purpose-built file system for handling large files and high-bandwidth workloads. We’ll break down how 3FS optimizes data access and speeds up AI workloads as well as the design tradeoffs made to maximize throughput for AI workloads.
This webinar you’ll learn about how 3FS works under the hood, including:
✅ The system architecture
✅ Core software components
✅ Read/write flows
✅ Data distribution/placement algorithms
✅ Cluster/node management and disaster recovery
Whether you’re an AI researcher, ML engineer, or infrastructure architect, this deep dive will give you the technical insights you need to determine if 3FS is the right solution for you.
.png)
Today’s conventional wisdom states that network latency across the two ends of a hybrid cloud prevents you from running analytic workloads in the cloud with the data on-prem. As a result, most companies copy their data into a cloud environment and maintain that duplicate data. All of this means that it is challenging to make both on-prem HDFS data accessible with the desired application performance.
In this talk, we will show you how to leverage any public cloud (AWS, Google Cloud Platform, or Microsoft Azure) to scale analytics workloads directly on on-prem data without copying and synchronizing the data into the cloud.
In this Office Hour, we will go over:
- A strategy to embrace the hybrid cloud, including an architecture for running ephemeral compute clusters using on-prem HDFS.
- An example of running on-demand Presto, Spark, and Hive with Alluxio in the public cloud.
- An analysis of experiments with TPC-DS to demonstrate the benefits of the given architecture.
Alluxio (alluxio.io) is an open-source data orchestration system that provides a single namespace federating multiple external distributed storage systems. It is critical for Alluxio to be able to store and serve the metadata of all files and directories from all mounted external storage both at scale and at speed.
This talk shares our design, implementation, and optimization of Alluxio metadata service (master node) to address the scalability challenges. Particularly, we will focus on how to apply and combine techniques including tiered metadata storage (based on off-heap KV store RocksDB), fine-grained file system inode tree locking scheme, embedded state-replicate machine (based on RAFT), exploration and performance tuning in the correct RPC frameworks (thrift vs gRPC) and etc. As a result of the combined above techniques, Alluxio 2.0 is able to store at least 1 billion files with a significantly reduced memory requirement, serving 3000 workers and 30000 clients concurrently.
In this Office Hour, we will go over how to:
- Metadata storage challenges
- How to combine different open source technologies as building blocks
- The design, implementation, and optimization of Alluxio metadata service
Presto, an open-source distributed SQL engine, is commonly used to query an existing Hive data warehouse. Due to existing applications, tech debt or operational challenges in the past, Presto may not be able to achieve its full potential but bound and limited by the past decisions. Particularly, challenges include overloaded Hive Metastore with slow and unpredictable access, unoptimized data formats and layouts such as too many small files, or lack of influence over the existing Hive system and other Hive applications.
Ideally, Presto would access data independently from how the data was originally stored or managed. Alluxio, as a data orchestration layer provides the physical data independence, for Presto to interact with the data more efficiently. In addition to caching for IO acceleration, Alluxio also provides a catalog service to abstract the metadata in the Hive Metastore, and transformations to expose the data in compute-optimized way. In this talk, we describe some of the challenges of using Presto with Hive, and introduce Alluxio data orchestration for solving those challenges.
In this Office Hour, we will go over:
- Typical challenges of using Presto with Hive
- Overview of the different services of Alluxio Structured Data Management in Alluxio 2.1
- A demo of using Alluxio Structured Data Management with Presto
Accessing data to run analytic workloads in Spark across data centers and/or clouds can be challenging. Additionally, network I/O can bottleneck Spark jobs that need to read a large amount of data. A common solution is to deploy an HDFS cluster closer to Spark as a caching layer and manually copy the input data to HDFS first, purging it afterward. But this ETL process can be both time-consuming and also error-prone.
A more efficient and simpler solution is to run Spark on Alluxio as a distributed cache on top of the remote data source. While caching data transparently based on access patterns and storing the working set closer, Alluxio provides Spark jobs much higher I/O throughput with enhanced data locality. In addition, Alluxio also provides data accessibility and abstraction for deployments in hybrid and multi-cloud environments.
In this Office Hour, we will go over how to:
- Burst on-prem Spark workloads to the cloud with Alluxio so Spark can seamlessly read from and write to remote data storage
- Use Alluxio as the input/output for Spark applications
- Save and load Spark RDDs and Dataframes with Alluxio
Building distributed systems is no small feat. Software testing is just one of many critical practices that engineers who build these systems need to utilize to ensure the quality and usability of their software. For distributed systems, scaling out testing frameworks to ensure that enterprises who run our in highly distributed environments is a complicated (and expensive task!)
In this online meetup, you will learn about:
- How the engineers at Alluxio have approached testing at scale
- Approaches to maintaining distributed systems at scale
Many organizations are leveraging EMR to run big data analytics on public cloud. However, reading and writing data to S3 directly can result in slow and inconsistent performance. Alluxio is a data orchestration layer for the cloud, and in this use case it caches data for S3, ensuring high and predictable performance as well as reduced network traffic.
In this office hour, you will learn about:
- How to set up Alluxio with the EMR stack so that Presto jobs can seamlessly read from and write to S3
- Compare the performance between Presto on EMR with Presto and Alluxio on EMR
- Open Session for discussion on any topics such as solving the separation of compute and storage problem, and more
With the advent of the public clouds and data increasingly siloed across many locations — on premises and in the public cloud — enterprises are looking for more flexibility and higher performance approaches to analyze their structured data.
Join us for this tech talk where we’ll introduce the Starburst Presto, Alluxio, and cloud object store stack for building a highly-concurrent and low-latency analytics platform. This stack provides a strong solution to run fast SQL across multiple storage systems including HDFS, S3, and others in public cloud, hybrid cloud, and multi-cloud environments. You’ll learn more about:
- The architecture of Presto, an open source distributed SQL engine
- How the Presto + Alluxio stack queries data from cloud object storage like S3 for faster and more cost-effective analytics
- Achieving data locality and cross-job caching with Alluxio regardless of where data is persisted
Data scientists or platform engineers often face the following challenge when the input data for machine learning jobs are stored in remote storage like NFS or cloud storage like S3. Making direct data access is slow, unstable and expensive; manually duplicating data to the training clusters also introduces large overhead, complicated data curation and often requires engineers to build ETL pipelines.
This talk will guide the audience on how Alluxio can greatly simplify the data preparation phase in with remote and possibly multiple data sources. We will share the lessons and benchmark from Bill Zhao an engineer led in Apple when building a Machine Learning platform using Tensorflow, NFS, DC/OS and Alluxio.
In this online meetup, you will learn about:
- When Alluxio can help for machine learning platform;
- How to setup and create POSIX endpoint for Alluxio service to unify the file system data access to S3, HDFS and Azure blob storage;
- How to run TensorFlow to train models backed by accessing remote input data like access local file system.
Users deploy Alluxio in a wide range of use cases from analytics to AI platforms, for Alluxio’s unified access to data and transparent caching for acceleration. However, many frameworks are SQL engines, like Presto, Apache Spark SQL, or Apache Hive, and consume data structured as tables of rows and columns. Since Alluxio is commonly used as a filesystem of files and directories, there is a mismatch between how Alluxio exposes data (files, directories), and how SQL engines deal with data (tables, rows, columns). This gap creates various challenges and inefficiencies.
Therefore, in the Alluxio 2.1 release, we introduce Alluxio Structured Data Management, which is a new set of services that enables structured data applications to interact with data more efficiently. The new services include the catalog service and a transformation service, which all work together to bridge the gap between storage and SQL engines and enable physical data independence.
In this office hour, we introduce the concepts and components of Alluxio Structured Data Management, and go through a demo with Presto.
In this Office Hour we’ll go over:
- Introduction and motivation of Alluxio Structured Data Management
- Overview of the different services of Alluxio Structured Data Management in Alluxio 2.1
- A demo of using Alluxio Structured Data Management with Presto
While adoption of the Cloud & Kubernetes has made it exceptionally easy to scale compute, the increasing spread of data across different systems and clouds has created new challenges for data engineers. Effectively accessing data from AWS S3 or on-premises HDFS becomes harder and data locality is also lost – how do you move data to compute workers efficiently, how do you unify data across multiple or remote clouds, and many more. Open source project Alluxio approaches this problem in a new way. It helps elastic compute workloads, such as Apache Spark, realize the true benefits of the cloud while bringing data locality and data accessibility to workloads orchestrated by Kubernetes.
One important performance optimization in Apache Spark is to schedule tasks on nodes with HDFS data nodes locally serving the task input data. However, more users are running Apache Spark natively on Kubernetes where HDFS is not an option. This office hour describes the concept and dataflow with respect to using the stack of Spark/Alluxio in Kubernetes with enhanced data locality even if the storage service is outside or remote.
In this Office Hour we’ll go over:
- Why Spark is able to make a locality-aware schedule when working with Alluxio in K8s environment using the host network
- Why a pod running Alluxio can share data efficiently with a pod running Spark on the same host using domain socket and host path volume
- The roadmap to improve this Spark / Alluxio stack in the context of K8s
Google Cloud Dataproc is a widely used fully managed Spark and Hadoop service to run big data analytics and compute workloads in the cloud. Services like Dataproc reduce hardware spend, eliminate the need to overbuy capacity, and provide business agility. Yet users still face challenges for performance sensitive workloads or workloads running on remote data.
Alluxio is an open source cloud data orchestration platform that increases performance of analytic workloads running on Dataproc by intelligently caching data and bringing back lost data locality. Alluxio also enables users to run compute workloads against on-prem storage like Hadoop HDFS without any app changes.
Chris Crosbie and Roderick Yao from the Google Dataproc team and Dipti Borkar of Alluxio demo how to set up Google Cloud Dataproc with Alluxio so jobs can seamlessly read from and write to Cloud Storage. They also show how to run Dataproc Spark against a remote HDFS cluster.
If you’re a MapR user, you might have concerns with your existing data stack. Whether it’s the complexity of Hadoop, financial instability and no future MapR product roadmap, or no flexibility when it comes to co-locating storage and compute, MapR may no longer be working for you.
Alluxio can help you migrate to a modern, disaggregated data stack using any object store with the similar performance of Hadoop plus significant cost savings.
Join us for this tech talk where we’ll discuss how to separate your compute and storage on-prem and architect a new data stack that makes your object store the core. We’ll show you how to offload your MapR/HDFS compute to any object store and how to run all of your existing jobs as-is on Alluxio + object store.