On-Demand Videos
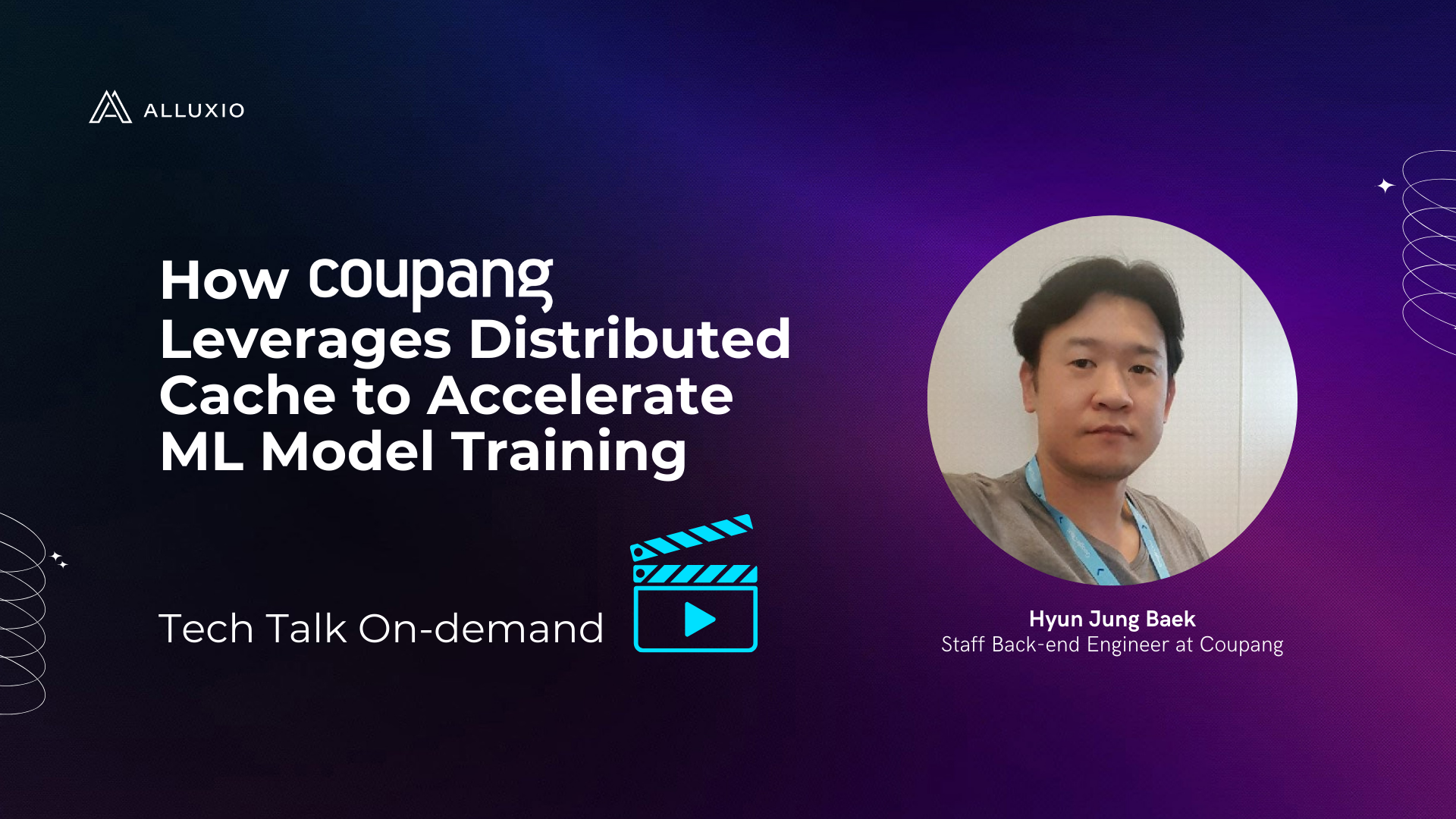
Coupang is a leading e-commerce company in South Korea, with over 50,000 employees and $20+ billion in annual revenue. Coupang's AI platform team builds and manages a large-scale AI platform in AWS for machine learning engineers to train models that enhance and customize product search results and product recommendations for its 100+ million customers.
As the search and recommendation models evolve, optimizing the underlying infrastructure for AI/ML workloads is essential for the e-commerce business. Coupang's platform team actively sought to improve their model training pipeline to boost machine learning engineers' productivity, publish models to production faster, and reduce operational costs.
Coupang focused on addressing several key areas:
- Shortening data preparation and model training time
- Improving GPU utilization in training clusters in different regions
- Reducing S3 API and egress costs incurred from copying large training datasets across regions
- Simplifying the operational complexity of storage system management
In this tech talk, Hyun Jung Baek, Staff Backend Engineer at Coupang, will share best practices for leveraging distributed caching to power search and recommendation model training infrastructure.
Hyun will discuss:
- How Coupang builds a world-class large-scale AI platform for machine learning engineers to deliver better search and recommendation models
- How adding distributed caching to their multi-region AI infrastructure improves GPU utilization, accelerates end-to-end training time, and significantly reduces cross-region data transfer costs.
- How to simplify platform operations and to easily deploy the same architecture to new GPU clusters.
About the Speaker
Hyun Jung Baek is a Staff Backend Engineer at Coupang.
Deepseek’s recent announcement of the Fire-flyer File System (3FS) has sparked excitement across the AI infra community, promising a breakthrough in how machine learning models access and process data.
In this webinar, an expert in distributed systems and AI infrastructure will take you inside Deepseek 3FS, the purpose-built file system for handling large files and high-bandwidth workloads. We’ll break down how 3FS optimizes data access and speeds up AI workloads as well as the design tradeoffs made to maximize throughput for AI workloads.
This webinar you’ll learn about how 3FS works under the hood, including:
✅ The system architecture
✅ Core software components
✅ Read/write flows
✅ Data distribution/placement algorithms
✅ Cluster/node management and disaster recovery
Whether you’re an AI researcher, ML engineer, or infrastructure architect, this deep dive will give you the technical insights you need to determine if 3FS is the right solution for you.
.png)
Apache Spark has been widely adopted for in-memory data analytics at scale, however, efficient memory utilization is a common challenge, and users will either run out of memory or experience low and unstable performance. Many Spark users may not be aware of the differences in memory utilization between caching data directly in-memory into the Spark JVM versus storing data off-heap via an in-memory storage service like Alluxio. In this office hour, I will highlight the two approaches with a demo and open up for discussions
In this Office Hour we’ll go over:
- How to run Spark shell with Alluxio such that Spark jobs
- A demo to compare the memory usage between Spark cache and using Alluxio as the external off-heap caching service
- Open Session for discussion on any topics such as running Presto on Alluxio, and more
The DBS team was tasked to solve their compute capacity problem. They wanted to provide faster insights and analyze data for a range of use cases but didn’t have the ability to scale compute elastically on-prem.
One use case that challenged them was customer call analysis. With the millions of customer calls they get every year, DBS manages over 50TB of customer data and audio files. This data needed to reside on-prem for compliance reasons. With on-prem compute limitations, they looked to the public cloud to analyze this data and selected “zero-copy” bursting as the best approach.
In this tech talk, we’ll discuss why DBS turned to Alluxio’s bursting approach to help solve these challenges. Vitaliy Baklikov, SVP at DBS, will discuss:
- Challenges and inefficiencies with their prior data stack
- Moving to a disaggregated data stack using Alluxio
- Bursting data without persisting in the cloud
- An overview of Alluxio’s “zero-copy” hybrid bursting solution
tf.data is the recommended API for creating TensorFlow input pipelines and is relied upon by countless external and internal Google users. The API enables you to build complex input pipelines from simple, reusable pieces and makes it possible to handle large amounts of data, different data formats, and perform complex transformations. In this talk, I will present an overview of the project and highlight best practices for creating performant input pipelines.
Apache Iceberg is a new format for tracking very large scale tables that are designed for object stores like S3. This talk will include why Netflix needed to build Iceberg, the project’s high-level design, and will highlight the details that unblock better query performance. Apache Iceberg – A Table Format for Hige Analytic Datasets from Alluxio, Inc.
In this keynote, Haoyuan will discuss the key challenges and trends impacting data engineering, and explore the concept of Data Orchestration.
Deep Learning and Gene Computing Acceleration with Alluxio in Kubernetes from Alluxio, Inc.
Alluxio Innovations for Structured Data from Alluxio, Inc.
The Data Flywheel is a comprehensive and additive approach for business and technology leaders to enable organizations to get the most value from their data. In this session, we will share common design patterns AWS customers are applying as part of their Data and AI journey. It will include real world examples. Modern Data Platforms – Thinking Data Flywheel on the Cloud from Alluxio, Inc.
Challenge And Evolution Of Data Orchestration at Rakuten Data System from Alluxio, Inc.
At Ryte, we analyze unstructured, semi-structured and structured data for more than one million users worldwide. The whole Ryte-Platform is built with a scalable architecture to support our heavy load and make it possible for our customers to drill-down from a high-level overview into the last byte of their websites. Presto + Alluxio on steroids a romantic drama on Production with happy end from Alluxio, Inc.
Alluxio core maintainers and founding engineers share the latest innovations in Alluxio 2. Alluxio 2 Community Update from Alluxio, Inc.
Presto, an open source distributed SQL engine, is widely recognized for its low-latency queries, high concurrency, and native ability to query multiple data sources. Proven at scale in a variety of use cases at Airbnb, Comcast, GrubHub, Facebook, FINRA, LinkedIn, Lyft, Netflix, Twitter, and Uber, in the last few years Presto experienced an unprecedented growth in popularity in both on-premises and cloud deployments over Object Stores, HDFS, NoSQL and RDBMS data stores.
This talk will discuss best use cases for Presto from the Data Engineer’s perspective. In addition, we will present the recent Presto advancements such as Cost-Based Optimizer, Kubernetes-native deployment and the project roadmap going forward.