On-Demand Videos
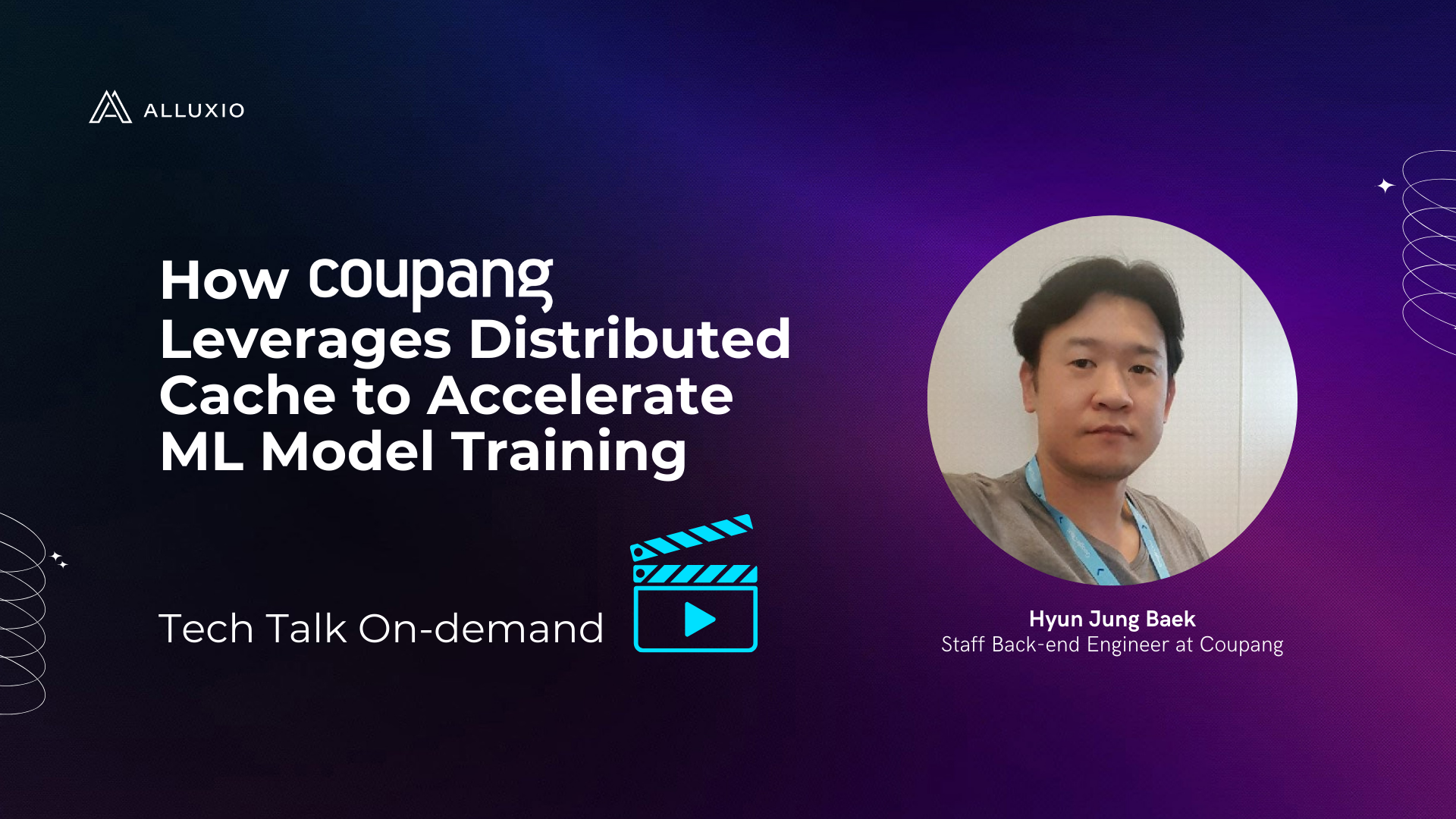
Coupang is a leading e-commerce company in South Korea, with over 50,000 employees and $20+ billion in annual revenue. Coupang's AI platform team builds and manages a large-scale AI platform in AWS for machine learning engineers to train models that enhance and customize product search results and product recommendations for its 100+ million customers.
As the search and recommendation models evolve, optimizing the underlying infrastructure for AI/ML workloads is essential for the e-commerce business. Coupang's platform team actively sought to improve their model training pipeline to boost machine learning engineers' productivity, publish models to production faster, and reduce operational costs.
Coupang focused on addressing several key areas:
- Shortening data preparation and model training time
- Improving GPU utilization in training clusters in different regions
- Reducing S3 API and egress costs incurred from copying large training datasets across regions
- Simplifying the operational complexity of storage system management
In this tech talk, Hyun Jung Baek, Staff Backend Engineer at Coupang, will share best practices for leveraging distributed caching to power search and recommendation model training infrastructure.
Hyun will discuss:
- How Coupang builds a world-class large-scale AI platform for machine learning engineers to deliver better search and recommendation models
- How adding distributed caching to their multi-region AI infrastructure improves GPU utilization, accelerates end-to-end training time, and significantly reduces cross-region data transfer costs.
- How to simplify platform operations and to easily deploy the same architecture to new GPU clusters.
About the Speaker
Hyun Jung Baek is a Staff Backend Engineer at Coupang.
Deepseek’s recent announcement of the Fire-flyer File System (3FS) has sparked excitement across the AI infra community, promising a breakthrough in how machine learning models access and process data.
In this webinar, an expert in distributed systems and AI infrastructure will take you inside Deepseek 3FS, the purpose-built file system for handling large files and high-bandwidth workloads. We’ll break down how 3FS optimizes data access and speeds up AI workloads as well as the design tradeoffs made to maximize throughput for AI workloads.
This webinar you’ll learn about how 3FS works under the hood, including:
✅ The system architecture
✅ Core software components
✅ Read/write flows
✅ Data distribution/placement algorithms
✅ Cluster/node management and disaster recovery
Whether you’re an AI researcher, ML engineer, or infrastructure architect, this deep dive will give you the technical insights you need to determine if 3FS is the right solution for you.
.png)
Today, one can easily launch or terminate services with hundreds or thousands of compute instances in just a few seconds on cloud services such as AWS. However, operating, monitoring and maintaining those resources could also easily become a nightmare if the corresponding systems were not designed in a cloud-native way.
In this talk, we share our lessons in building and rebuilding our monitoring systems and data platforms at Electronic Arts (EA). In the first generation of the monitoring system, configurations were manually created for many individual software components and spread over all the resources. As services were started and terminated rapidly over time, it was extremely difficult to keep all configurations up to date. Consequently, on average we received over 1,000 alerts from thousands of machines on a daily basis, which stressed the operations team. We redesigned the system in late 2018 in a project called Monitoring As Code (MAC) emphasizing on version control and automation. MAC manages all the configurations using a GIT project in the same way as software code. Moreover, it establishes standards so that the configurations are automatically generated and deployed to keep everything in sync. As a result, it reduced the daily average number of alerts by two orders of magnitude.
In the first generation of the data platform, we used HDFS as a cache layer between ETL jobs and the underlying AWS storage service S3. However, HDFS is not a special-purpose cache service, so custom code is needed to make it work like a cache. We have to run a backup workflow in every ETL job to backup data to S3 and sync the metadata store of the ETL jobs running on HDFS and that of interactive analytic queries running directly on S3. Moreover, we rely on complex and fragile mechanisms for purging datasets when the clusters are under heavy load. The use of HDFS also makes it a challenge to rapidly scale up the YARN cluster during peak hours and scale it down during off-hours. We are currently redesigning the data platform, mainly by replacing HDFS with a special-purpose data orchestration service called Alluxio. In our initial evaluation, Alluxio not only provides better performance than HDFS but also significantly simplifies the architecture of our data platform and makes it easy to scale up and down and paves the way to a cloud native ETL processing stack.
This DATA ORCHESTRATION SUMMIT session talks about challenges associated with querying diverse data sources at Walmart and how those are tackled using Presto & Alluxio.
How Alluxio caching was leveraged to provide consistent optimized query performance within and across clouds.
Also highlights implementation of critical components for Enterprise acceleration offering such as security integration for fine grained access control, auto-scaling & auto deployment in GCP.
In this panel, creators of open source projects share their stories from why they started the project to the challenges they encountered on the way.
Spark is a widely adopted open source framework that provides a unified interface for analytics and machine learning workloads. Alluxio, originating from the UC Berkeley AMPLab – the same lab as Spark, is an open source data orchestration platform that empowers compute frameworks like Spark by providing stateful caching to enable efficient data sharing between multiple jobs and improving resilience against job failures as well as bringing data together from many different sources, be it remote HDFS or cloud object stores.
Alluxio partnered with IBM to deliver a Spark-based solution to provide fast data analytics. With the integration of IBM Spectrum Conductor, an advanced workload and resource management platform that maximizes hardware utilization to speed results and cut infrastructure costs, Alluxio and IBM delivered a solution that powers leading telecom company’s applications to support 320 million subscribers. In this online meetup, we will present the benefits of the fast analytics stack of Spark on Alluxio and IBM and dive into a leading telecom’s use case of leveraging Spark and Alluxio to process massive amounts of mobile data.
In this online meetup, you will learn about:
- Why the leading companies are moving towards a decoupled compute and storage architecture, and the associated challenges and requirements.
- Why Spark and Alluxio together can solve the challenges and fulfill the requirements
- How leading telecom leverages Spark with Alluxio for fast data processing at scale on top of object store and HDFS
Using “zero-copy” hybrid bursting with Spark to solve capacity problems
Want to leverage your existing investments in Hadoop with your data on-premise and still benefit from the elasticity of the cloud?
Like other Hadoop users, you most likely experience very large and busy Hadoop clusters, particularly when it comes to compute capacity. Bursting HDFS data to the cloud can bring challenges – network latency impacts performance, copying data via DistCP means maintaining duplicate data, and you may have to make application changes to accomodate the use of S3.
“Zero-copy” hybrid bursting with Alluxio keeps your data on-prem and syncs data to compute in the cloud so you can expand compute capacity, particularly for ephemeral Spark jobs.
In this tech talk, we’ll discuss:
- Approaches to burst data to the cloud
- How Alluxio can enable “zero-copy” bursting of Spark workloads to cloud data services like EMR and Dataproc
- How DBS Bank uses Alluxio to solve for limited on-prem compute capacity by zero-copy bursting Spark workloads to AWS EMR
The data ecosystem has heavily evolved over the past two decades. There’s been an explosion of data-driven frameworks, such as Presto, Hive, and Spark to run analytics and ETL queries and TensorFlow and PyTorch to train and serve models. On the data side, the approach to managing and storing data has evolved from HDFS to cheaper, more scalable and separated services typified by cloud stores like AWS S3. As a result, data engineering has become increasingly complex, inefficient, and hard, particularly in hybrid and cloud environments.
Haoyuan Li offers an overview of a data orchestration layer that provides a unified data access and caching layer for single cloud, hybrid, and multicloud deployments. It enables distributed compute engines like Presto, TensorFlow, and PyTorch to transparently access data from various storage systems (including S3, HDFS, and Azure) while actively leveraging an in-memory cache to accelerate data access.
Many organizations are leveraging Hive to run big data analytics on public cloud. However, reading and writing data to S3 directly can result in slow and inconsistent performance. Alluxio is a data orchestration layer for the cloud, and in this use case it caches data for S3, ensuring high and predictable performance as well as reduced network traffic.
In this Office Hour we’ll go over:
- Bazaarvoice’s use case leveraging Apache Spark, Hive, and Alluxio on S3
- How to set up Hive with Alluxio such that Hive jobs can seamlessly read from and write to S3
- Open Session for discussion on any topics such as solving the separation of compute and storage problem, and more
ING Bank is a multinational financial services company headquartered in Amsterdam with over $1 trillion in assets. As a leading bank, we place a great emphasis on cybersecurity. One aspect of this is the Security incident and event management (SIEM), which is the process of identifying, monitoring, recording and analyzing security events or incidents within a real-time IT environment. SIEM requires our data platform to have high and consistent performance, so we use open source technologies Presto and Alluxio for fast SQL analytics in the cloud.
In this online presentation, we are going to present how ING is leveraging Presto (interactive query), Alluxio (data orchestration & acceleration), S3 (massive storage), and DC/OS (container orchestration) to build and operate our modern Security Analytics & Machine Learning platform. We will share the challenges we encountered and how we solved them. Today we run this platform in several different data centers, and we have reduced our 10+ minutes queries to under 10 seconds!
EMR has become a widely used service to run big data analytics in the public cloud. But issues around slow/inconsistent EMR performance due to S3 data lakes creates challenges for organizations.
Alluxio is a data orchestration layer for the cloud that increases performance of analytic workloads running on AWS EMR using S3 as the storage.
Join us for this webinar where we will show you how to set up EMR Spark and Hive with Alluxio so jobs can seamlessly read from and write to your S3 data lake. You’ll see the performance gains with Alluxio in your EMR/S3 stack.
Many organizations are leveraging EMR to run big data analytics on public cloud. However, reading and writing data to S3 directly can result in slow and inconsistent performance. Alluxio is a data orchestration layer for the cloud, and in this use case it caches data for S3, ensuring high and predictable performance as well as reduced network traffic.
In this Office Hour we go over:
- How to set up EMR Spark with Alluxio such that Spark jobs can seamlessly read from and write to S3
- Compare the performance between Spark on S3 with Spark and Alluxio on S3
- Open Session for discussion on any topics such as solving the separation of compute and storage problem, and more
Kubernetes is widely used across enterprises to orchestrate computation. And while Kubernetes helps improve flexibility and portability for computation in public/hybrid cloud environments across infrastructure providers, running data-intensive workloads can be challenging.
When it comes to efficiently moving data closer to Spark or Presto frameworks, co-locating data with these frameworks and accessing data from multiple or remote clouds is hard to do. That’s where Alluxio, an open source data orchestration platform, can help.
Alluxio enables data locality with your Spark and Presto workloads for faster performance and better data accessibility in Kubernetes. It also provides portability across storage providers.
In this on demand tech talk we’ll give a quick overview of Alluxio and the use cases it powers for Spark/Presto in Kubernetes. We’ll show you how to set up Alluxio and Spark/Presto to run in Kubernetes as well.
Alluxio 2.0 is the most ambitious platform upgrade since the inception of Alluxio with greatly expanded capabilities to empower users to run analytics and AI workloads on private, public or hybrid cloud infrastructures leveraging valuable data wherever it might be stored.
This release, now available for download, includes many advancements that will allow users to push the limits of their data-workloads in the cloud.
In this tech talk, we will introduce the key new features and enhancements such as:
- Support for hyper-scale data workloads with tiered metadata storage, distributed cluster services, and adaptive replication for increased data locality
- Machine learning and deep learning workloads on any storage with the improved POSIX API
- Better storage abstraction with support for HDFS clusters across different versions & active sync with Hadoop