On-Demand Videos
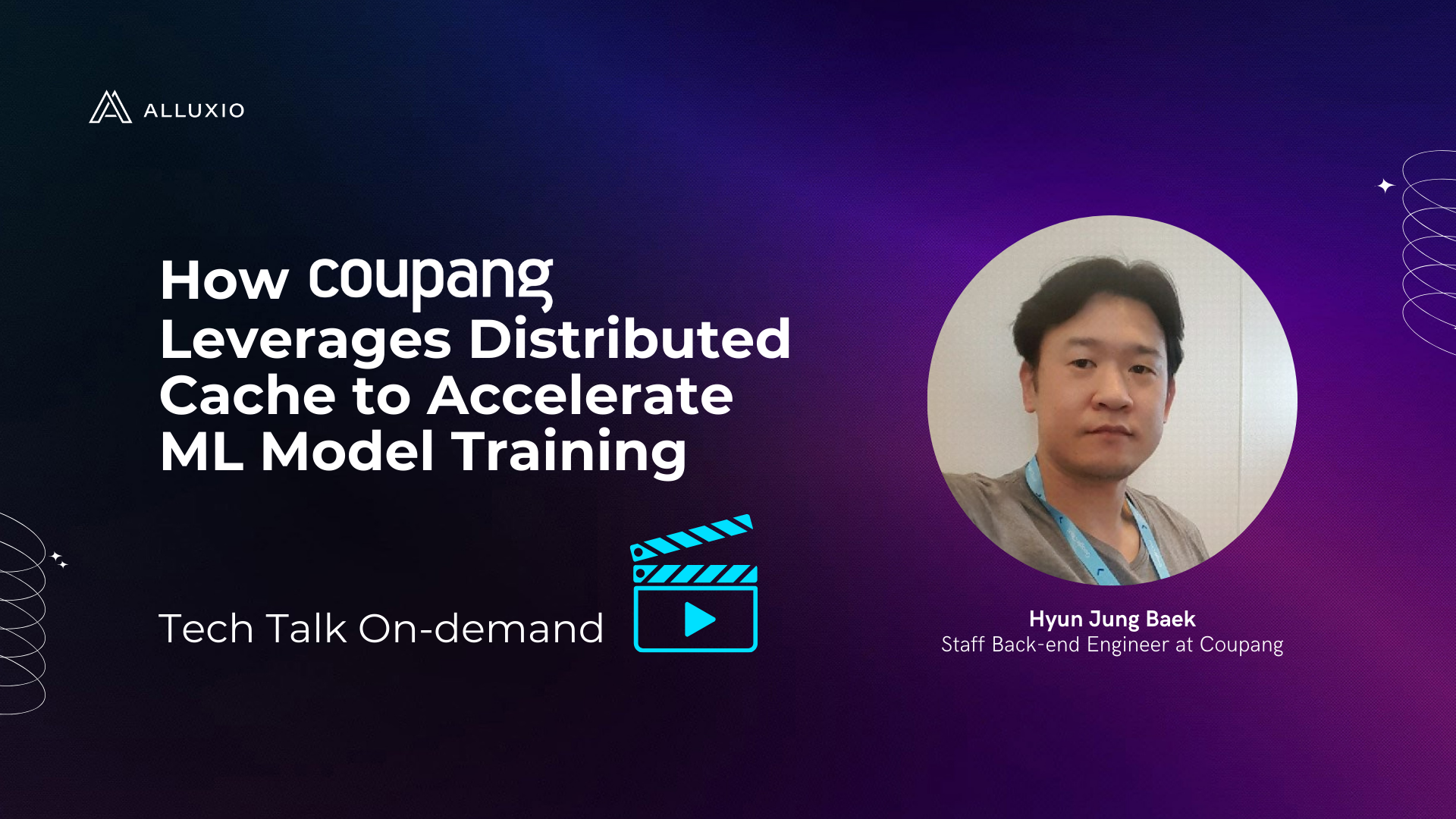
Coupang is a leading e-commerce company in South Korea, with over 50,000 employees and $20+ billion in annual revenue. Coupang's AI platform team builds and manages a large-scale AI platform in AWS for machine learning engineers to train models that enhance and customize product search results and product recommendations for its 100+ million customers.
As the search and recommendation models evolve, optimizing the underlying infrastructure for AI/ML workloads is essential for the e-commerce business. Coupang's platform team actively sought to improve their model training pipeline to boost machine learning engineers' productivity, publish models to production faster, and reduce operational costs.
Coupang focused on addressing several key areas:
- Shortening data preparation and model training time
- Improving GPU utilization in training clusters in different regions
- Reducing S3 API and egress costs incurred from copying large training datasets across regions
- Simplifying the operational complexity of storage system management
In this tech talk, Hyun Jung Baek, Staff Backend Engineer at Coupang, will share best practices for leveraging distributed caching to power search and recommendation model training infrastructure.
Hyun will discuss:
- How Coupang builds a world-class large-scale AI platform for machine learning engineers to deliver better search and recommendation models
- How adding distributed caching to their multi-region AI infrastructure improves GPU utilization, accelerates end-to-end training time, and significantly reduces cross-region data transfer costs.
- How to simplify platform operations and to easily deploy the same architecture to new GPU clusters.
About the Speaker
Hyun Jung Baek is a Staff Backend Engineer at Coupang.
Deepseek’s recent announcement of the Fire-flyer File System (3FS) has sparked excitement across the AI infra community, promising a breakthrough in how machine learning models access and process data.
In this webinar, an expert in distributed systems and AI infrastructure will take you inside Deepseek 3FS, the purpose-built file system for handling large files and high-bandwidth workloads. We’ll break down how 3FS optimizes data access and speeds up AI workloads as well as the design tradeoffs made to maximize throughput for AI workloads.
This webinar you’ll learn about how 3FS works under the hood, including:
✅ The system architecture
✅ Core software components
✅ Read/write flows
✅ Data distribution/placement algorithms
✅ Cluster/node management and disaster recovery
Whether you’re an AI researcher, ML engineer, or infrastructure architect, this deep dive will give you the technical insights you need to determine if 3FS is the right solution for you.
.png)
We are excited to present Alluxio 2.0 to our community. The goal of Alluxio 2.0 was to significantly enhance data accessibility with improved APIs, expand use cases supported to include active workloads as well as better metadata management and availability to support hyperscale deployments. Alluxio 2.0 Preview Release is the first major milestone on this path to Alluxio 2.0 and includes many new features.
In this talk, I will give an overview of the motivations and design decisions behind the major changes in the Alluxio 2.0 release. We will touch on the key features:
– New off-Heap metadata storage leveraging embedded RocksDB to scale up Alluxio to handle a billion files;
– Improved Alluxio POSIX API to support legacy and machine-learning workloads;
– A fully contained, distributed embedded journal system based on RAFT consensus algorithm in high availability mode;
– A lightweight distributed compute framework called “Alluxio Job Service” to support Alluxio operations such as active replication, async-persist, cross mount move/copy and distributed loading;
– Support for mounting and connecting to any number of HDFS clusters of different versions at the same time;
Active file system sync between Alluxio and HDFS as under storage.
TFiR – Open Source & Emerging Technologies
In this interview we spoke to Haoyuan (H.Y.) Li, Founder, Chairman and CTO of Open Source Alluxio, a company that is democratizing data in the cloud.
The ever increasing challenge to process and extract value from exploding data with AI and analytics workloads makes a memory centric architecture with disaggregated storage and compute more attractive. This decoupled architecture enables users to innovate faster and scale on-demand. Enterprises are also increasingly looking towards object stores to power their big data & machine learning workloads in a cost-effective way. However, object stores don’t provide big data compatible APIs as well as the required performance.
In this webinar, the Intel and Alluxio teams will present a proposed reference architecture using Alluxio as the in-memory accelerator for object stores to enable modern analytical workloads such as Spark, Presto, Tensorflow, and Hive. We will also present a technical overview of Alluxio.
Interested in learning more?
While running analytics workloads using EMR Spark on S3 is a common deployment today, many organizations face issues in performance and consistency. EMR can be bottlenecked when reading large amounts of data from S3, and sharing data across multiple stages of a pipeline can be difficult as S3 is eventually consistent for read-your-own-write scenarios.
A simple solution is to run Spark on Alluxio as a distributed cache for S3. Alluxio stores data in memory close to Spark, providing high performance, in addition to providing data accessibility and abstraction for deployments in both public and hybrid clouds.
In this webinar you’ll learn how to:
- Increase performance by setting up Alluxio so Spark can seamlessly read from and write to S3
- Use Alluxio as the input/output for Spark applications
- Save and load Spark RDDs and Dataframes with Alluxio
Alluxio Open Source creator Haoyuan Li‘s keynote at O’Reilly Artificial Intelligence Conference discusses data revolution trend, the inevitable journey of data silos, and the missing piece of the data world – Data Orchestration System!
The data ecosystem has heavily evolved over the past two decades. There’s been an explosion of data-driven frameworks, such as Presto, Hive, and Spark to run analytics and ETL queries and TensorFlow and PyTorch to train and serve models. On the data side, the approach to managing and storing data has evolved from HDFS to cheaper, more scalable and separated services typified by cloud stores like AWS S3. As a result, data engineering has become increasingly complex, inefficient, and hard, particularly in hybrid and cloud environments.
Haoyuan Li offers an overview of a data orchestration layer that provides a unified data access and caching layer for single cloud, hybrid, and multicloud deployments. It enables distributed compute engines like Presto, TensorFlow, and PyTorch to transparently access data from various storage systems (including S3, HDFS, and Azure) while actively leveraging an in-memory cache to accelerate data access.
Alluxio 2.0 is the most ambitious platform upgrade since the inception of Alluxio with greatly expanded capabilities to empower users to run analytics and AI workloads on private, public or hybrid cloud infrastructures leveraging valuable data wherever it might be stored. This preview release, now available for download, includes many advancements that will allow users to push the limits of their data-workloads in the cloud.
In this webinar, we will introduce the key new features and enhancements such as:
- Support for hyper-scale data workloads with tiered metadata storage, distributed cluster services, and adaptive replication for increased data locality
- Machine learning and deep learning workloads on any storage with the improved POSIX API
- Better storage abstraction with support for HDFS clusters across different versions & active sync with Hadoop
As data analytic needs have increased with the explosion of data, the importance of the speed of analytics and the interactivity of queries has increased dramatically
In this tech talk, we will introduce the Starburst Presto, Alluxio, and cloud object store stack for building a highly-concurrent and low-latency analytics platform. This stack provides a strong solution to run fast SQL across multiple storage systems including HDFS, S3, and others in public cloud, hybrid cloud, and multi-cloud environments.
You’ll learn about:
- The architecture of Presto, an open source distributed SQL engine, as well as innovations by Starburst like as it’s cost-based optimizer
- How Presto can query data from cloud object storage like S3 at high performance and cost-effectively with Alluxio
- How to achieve data locality and cross-job caching with Alluxio no matter where the data is persisted and reduce egress costs
In addition, we’ll present some real world architectures & use cases from internet companies like JD.com and NetEase.com running the Presto and Alluxio stack at the scale of hundreds of nodes.
Enterprises are increasingly looking towards object stores to power their big data & machine learning workloads in a cost-effective way. The combination of SwiftStack and Alluxio together, enables users to seamlessly move towards a disaggregated architecture. Swiftstack provides a massively parallel cloud object storage and multi-cloud data management system. Alluxio is a data orchestration layer, which sits between compute frameworks and storage systems and enables big data workloads to be deployed directly on SwiftStack. Alluxio provides data locality, accessibility and elasticity via its core innovations. With the Alluxio and Swiftstack solution, Spark, Presto, Tensorflow and Hive and other compute workloads can benefit from 10X performance improvement and dramatically lower costs. In this tech talk, we will provide a brief overview of the Alluxio and SwiftStack solution as well as the key use cases it enables.
You’ll learn about:
- The trends driving organizations towards object stores
- An overview of Swiftstack and Alluxio
- Deep dive into the benefits of the Swiftstack Data Analytics Solution with Alluxio
The rise of compute intensive workloads and the adoption of the cloud has driven organizations to adopt a decoupled architecture for modern workloads – one in which compute scales independently from storage. While this enables scaling elasticity, it introduces new problems – how do you co-locate data with compute, how do you unify data across multiple remote clouds, how do you keep storage and I/O service costs down and many more.
Enter Alluxio, a virtual unified file system, which sits between compute and storage that allows you to realize the benefits of a hybrid cloud architecture with the same performance and lower costs.
In this webinar, we will discuss:
- Why leading enterprises are adopting hybrid cloud architectures with compute and storage disaggregated
- The new challenges that this new paradigm introduces
- An introduction to Alluxio and the unified data solution it provides for hybrid environments