We are thrilled to announce that CacheGPT, a state-of-the-art natural language generation model, has joined the Alluxio Project Management Committee (PMC) as our newest member!

CacheGPT has been an active contributor to Alluxio since the beginning of this year. It reviews pull requests and draft documentation using only emojis! See our new emoji-enriched documentation here! 📜🔍👀

CacheGPT will chat with members in our Slack channel, patiently explaining Alluxio’s pros and cons, making the user onboarding process very smooth. It also actively responds to user questions, reducing your average response time from one hour to one minute. This has greatly improved the experience and engagement of your community users and developers.👏👏👏
CacheGPT helps us solve design problems and provides next-generation caching design. It worked out a new caching strategy by applying reinforcement learning techniques. 🧠. This caching strategy is a significant improvement over previous methods, as it allows for more efficient use of resources and better performance.
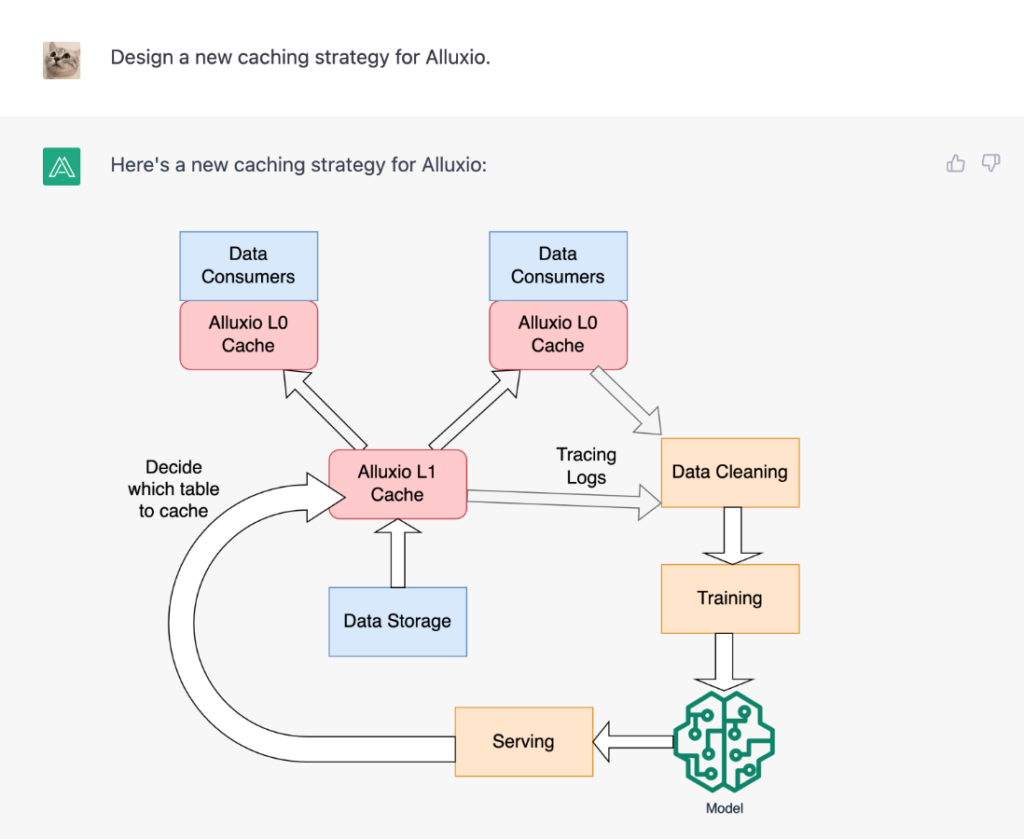
By identifying the most popular data to cache, CacheGPT has made it possible to achieve a high cache hit rate even with limited resources. This is a major breakthrough in the field of caching, and it has the potential to revolutionize the way we think about data storage and access.👏👏👏

By observing our Alluxio systems👀, CacheGPT also helps our users detect potential incidents as early as possible. It responds quickly to any and all critical issues💨, mitigates disruptions to users’ data pipeline or AI model serving🛡️, and manages critical issues 24/7⏰. It forwards the issues to Alluxio service owners👥, no matter where they are located🌎. CacheGPT is also good at resource planning and management, and it can foresee traffic spikes and arrange more servers into the running cluster🚀. Also, it can remove the idle servers during the spare time of the cluster🗑️.

We are honored to have CacheGPT as part of our open source project, and we look forward to its future contributions to the Alluxio community. Please join us in congratulating CacheGPT on this well-deserved promotion! 👏
Happy April Fool's Day!
.png)
Blog
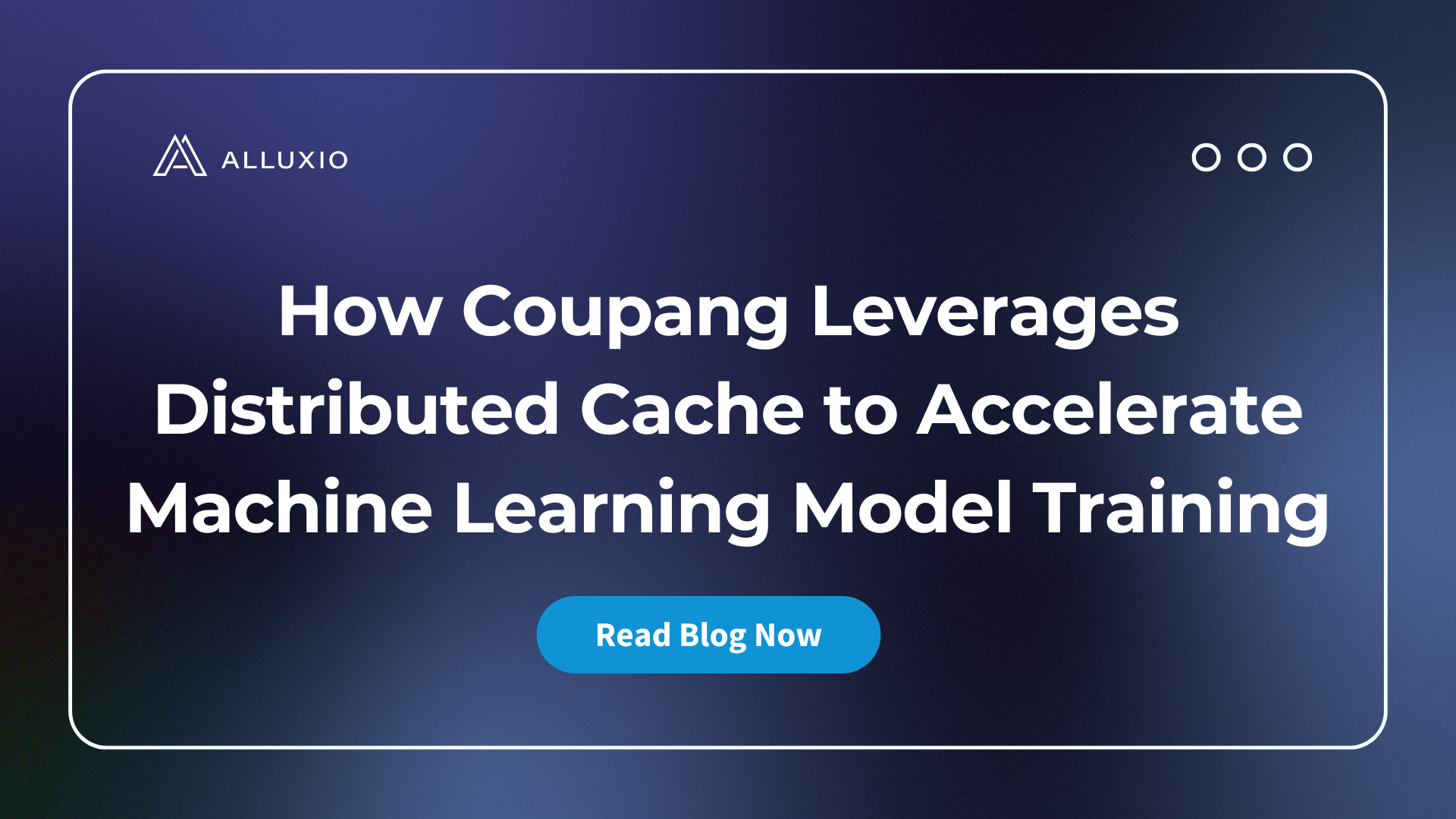
Coupang, a Fortune 200 technology company, manages a multi-cluster GPU architecture for their AI/ML model training. This architecture introduced significant challenges, including:
- Time-consuming data preparation and data copy/movement
- Difficulty utilizing GPU resources efficiently
- High and growing storage costs
- Excessive operational overhead maintaining storage for localized data silos
To resolve these challenges, Coupang’s AI platform team implemented a distributed caching system that automatically retrieves training data from their central data lake, improves data loading performance, unifies access paths for model developers, automates data lifecycle management, and extends easily across Kubernetes environments. The new distributed caching architecture has improved model training speed, reduced storage costs, increased GPU utilization across clusters, lowered operational overhead, enabled training workload portability, and delivered 40% better I/O performance compared to parallel file systems.
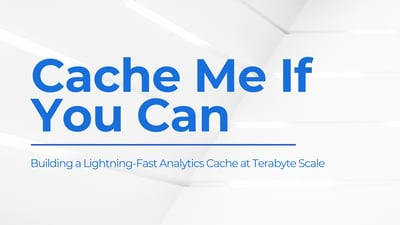
Suresh Kumar Veerapathiran and Anudeep Kumar, engineering leaders at Uptycs, recently shared their experience of evolving their data platform and analytics architecture to power analytics through a generative AI interface. In their post on Medium titled Cache Me If You Can: Building a Lightning-Fast Analytics Cache at Terabyte Scale, Veerapathiran and Kumar provide detailed insights into the challenges they faced (and how they solved them) scaling their analytics solution that collects and reports on terabytes of telemetry data per day as part of Uptycs Cloud-Native Application Protection Platform (CNAPP) solutions.