Alluxio is an open-source data orchestration system for analytics and AI workloads. Distributed applications like Apache Spark or Apache Hive can access Alluxio through its HDFS-compatible interface without code change. We refer to external storage such as HDFS or S3 as under storage.
Alluxio is a new layer on top of under storage systems that can not only improve raw I/O performance but also enables applications flexible options to read, write and manage files. This article focuses on describing different ways to write files to Alluxio, realizing the tradeoffs in performance, consistency, and also the level of fault tolerance compared to HDFS.
Given an application such as a Spark job which saves its output to an external storage service; Writing the job output to the memory layer in a colocated Alluxio worker will achieve the best write performance. Due to the volatility of memory, when a node in Alluxio goes down or restarts, any data in that node’s memory is lost.
To prevent data loss, Alluxio provides the ability to write the data to the persistent under storage either synchronously or asynchronously by configuring client-side Write Types. Each write type has benefits and drawbacks associated with it. Applications that write to Alluxio storage should consider the different write types and perform a cost-benefit analysis to determine the write type which is best-suited for the application requirements.
A summary of the available write types are listed below:
Write TypeDescriptionWrite SpeedFault ToleranceMUST_CACHEWrites directly to Alluxio memoryVery fastData loss if a worker crashesTHROUGHWrites directly to under storagelimited to under storage throughputDependent upon under storageCACHE_THROUGHWrites to Alluxio and under storage synchronouslyData in memory and persisted to under storage synchronouslyDependent upon under storageASYNC_THROUGHWrites to Alluxio first, then asynchronously writes to the under storageNearly as fast as MUST_CACHEand data persisted to under storage without user interactionPossible to lose data if only 1 replica is written
Write types are a client side property which means they can be modified when submitting the application without restarting any Alluxio processes. For example, to set the Alluxio write type to CACHE_THROUGH
when submitting a Spark job you can add the following options to the spark-submit
:
$ spark-submit \ --conf 'spark.driver.extraJavaOptions=-Dalluxio.user.file.writetype.default=CACHE_THROUGH' \ --conf 'spark.executor.extraJavaOptions=-Dalluxio.user.file.writetype.default=CACHE_THROUGH' \ ...
Here are some general bits of advice when choosing the right write type for your applications:
- For temporary data which doesn’t need to be saved or data that is very cheap to re-generate, use
MUST_CACHE
to write directly to Alluxio memory. It will then replicates over time, least safe, but most performant. - For data created that will not be used in the near term, use
THROUGH
to write it directly from the client application persisting immediately to the under storage, without caching another copy. This leaves more room in Alluxio storage for data which needs to be read fast and frequently. - For data must be persisted at the moment when the writer application returns, and will be used by other Alluxio applications very soon, use
CACHE_THROUGH
to both write data into Alluxio and the under storage. Note that, Alluxio may create replicas over time in Alluxio based on the data access pattern. - For data needs to be persisted and doesn’t need to be used immediately, use
ASYNC_THROUGH
which writes directly to Alluxio and then asynchronously persists data to the UFS.
.png)
Blog
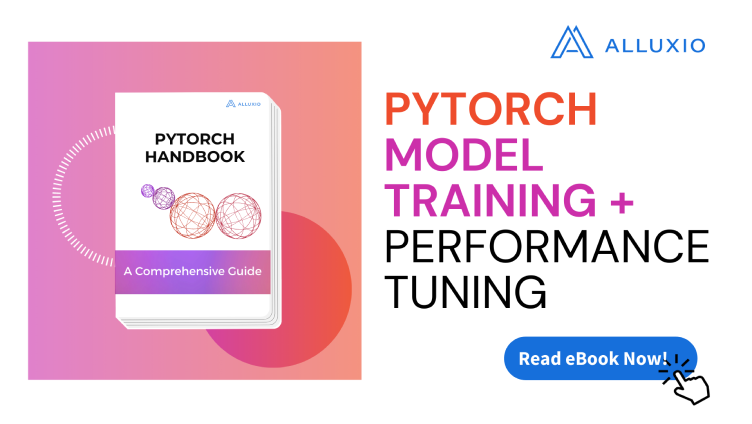
Coupang, a Fortune 200 technology company, manages a multi-cluster GPU architecture for their AI/ML model training. This architecture introduced significant challenges, including:
- Time-consuming data preparation and data copy/movement
- Difficulty utilizing GPU resources efficiently
- High and growing storage costs
- Excessive operational overhead maintaining storage for localized data silos
To resolve these challenges, Coupang’s AI platform team implemented a distributed caching system that automatically retrieves training data from their central data lake, improves data loading performance, unifies access paths for model developers, automates data lifecycle management, and extends easily across Kubernetes environments. The new distributed caching architecture has improved model training speed, reduced storage costs, increased GPU utilization across clusters, lowered operational overhead, enabled training workload portability, and delivered 40% better I/O performance compared to parallel file systems.
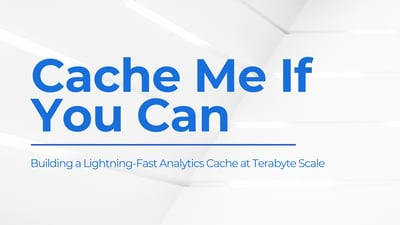
Suresh Kumar Veerapathiran and Anudeep Kumar, engineering leaders at Uptycs, recently shared their experience of evolving their data platform and analytics architecture to power analytics through a generative AI interface. In their post on Medium titled Cache Me If You Can: Building a Lightning-Fast Analytics Cache at Terabyte Scale, Veerapathiran and Kumar provide detailed insights into the challenges they faced (and how they solved them) scaling their analytics solution that collects and reports on terabytes of telemetry data per day as part of Uptycs Cloud-Native Application Protection Platform (CNAPP) solutions.