On-Demand Videos
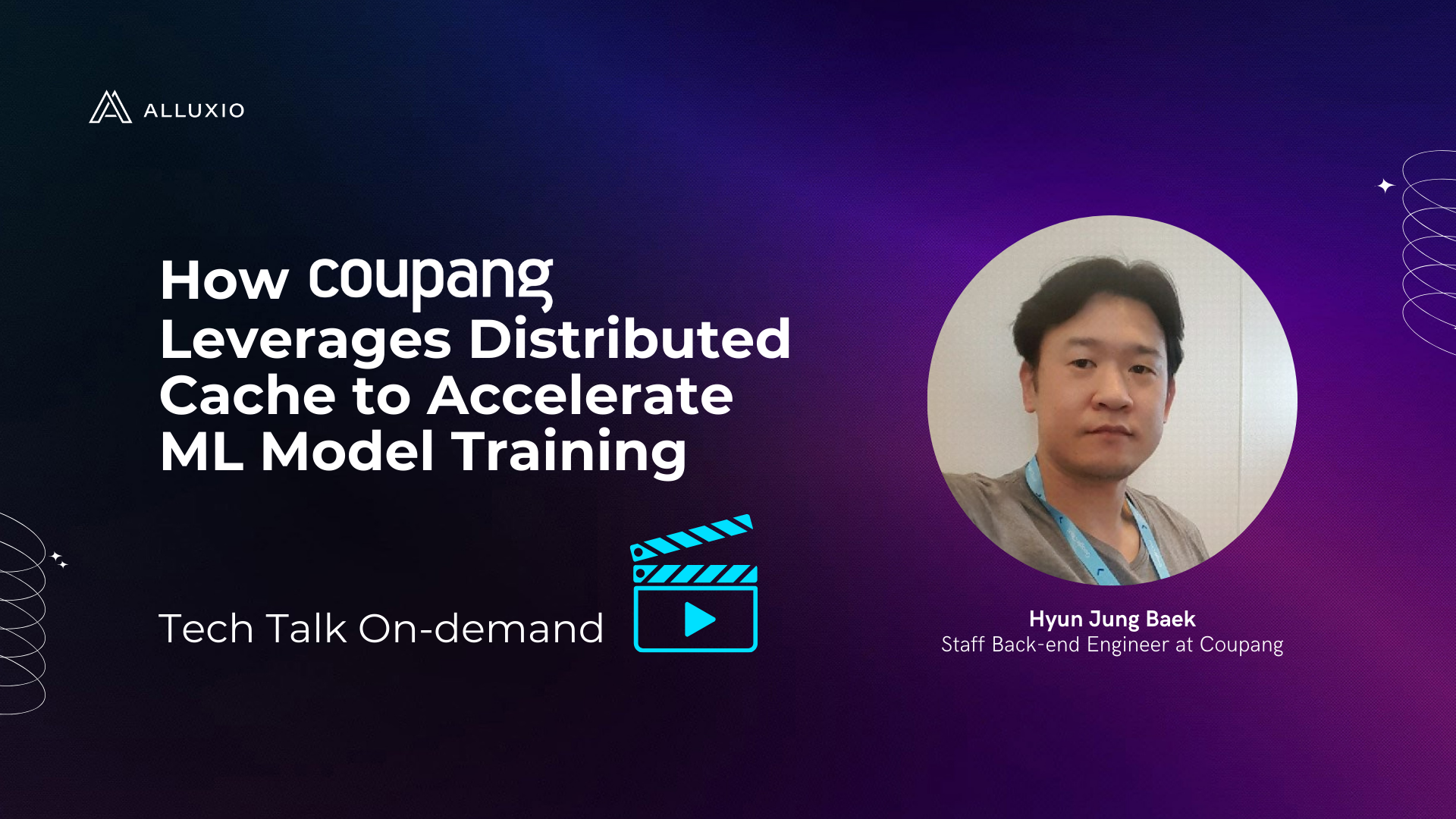
Coupang is a leading e-commerce company in South Korea, with over 50,000 employees and $20+ billion in annual revenue. Coupang's AI platform team builds and manages a large-scale AI platform in AWS for machine learning engineers to train models that enhance and customize product search results and product recommendations for its 100+ million customers.
As the search and recommendation models evolve, optimizing the underlying infrastructure for AI/ML workloads is essential for the e-commerce business. Coupang's platform team actively sought to improve their model training pipeline to boost machine learning engineers' productivity, publish models to production faster, and reduce operational costs.
Coupang focused on addressing several key areas:
- Shortening data preparation and model training time
- Improving GPU utilization in training clusters in different regions
- Reducing S3 API and egress costs incurred from copying large training datasets across regions
- Simplifying the operational complexity of storage system management
In this tech talk, Hyun Jung Baek, Staff Backend Engineer at Coupang, will share best practices for leveraging distributed caching to power search and recommendation model training infrastructure.
Hyun will discuss:
- How Coupang builds a world-class large-scale AI platform for machine learning engineers to deliver better search and recommendation models
- How adding distributed caching to their multi-region AI infrastructure improves GPU utilization, accelerates end-to-end training time, and significantly reduces cross-region data transfer costs.
- How to simplify platform operations and to easily deploy the same architecture to new GPU clusters.
About the Speaker
Hyun Jung Baek is a Staff Backend Engineer at Coupang.
Deepseek’s recent announcement of the Fire-flyer File System (3FS) has sparked excitement across the AI infra community, promising a breakthrough in how machine learning models access and process data.
In this webinar, an expert in distributed systems and AI infrastructure will take you inside Deepseek 3FS, the purpose-built file system for handling large files and high-bandwidth workloads. We’ll break down how 3FS optimizes data access and speeds up AI workloads as well as the design tradeoffs made to maximize throughput for AI workloads.
This webinar you’ll learn about how 3FS works under the hood, including:
✅ The system architecture
✅ Core software components
✅ Read/write flows
✅ Data distribution/placement algorithms
✅ Cluster/node management and disaster recovery
Whether you’re an AI researcher, ML engineer, or infrastructure architect, this deep dive will give you the technical insights you need to determine if 3FS is the right solution for you.
.png)
Driven by strong interests from our open source community, the Alluxio core engineering team re-designed things to come up with a more efficient and transparent way for users to leverage data orchestration through the POSIX interface. This enables much better performance for ML workloads where data is accessed via the POSIX interface.
In this 20 minute community session, you’ll hear from Lu Qiu, one of Alluxio’s lead engineers on the POSIX implementation project.
In this session, you’ll learn:
- How Alluxio’s new JNI-based FUSE implementation supports more efficient POSIX data access
- How improvements to multiple data operations, including distributedLoad, optimizations on listing or calculating directories with a massive amounts of files, etc., improve performance. In model training
- How these latest enhancements improve performance on TensorFlow and PyTorch training workloads, even with GPU-based training and compute
ALLUXIO DAY V 2021 August 27, 2021
ALLUXIO DAY V 2021 August 27, 2021
ALLUXIO DAY V 2021 August 27, 2021
ALLUXIO DAY V 2021 August 27, 2021
With data lakes expanding from on-prem to the cloud as well as increasing use of new object data stores, data platform teams are challenged with providing consistent, high-throughput access to distributed data sources for analytics and AI/ML applications. In today’s hybrid cloud and multi-cloud era, data-intensive applications such as Presto, Spark, Hive, and Tensorflow are suffering more sluggish response times and increased complexity with the growing separation of data and compute.
Join Alluxio’s distributed systems experts as they explore today’s data access challenges and open source data orchestration solutions for modernizing your data platform.
In this tech talk, you’ll learn:
- How data access and throughput challenges are hindering large-scale analytics and AI/ML applications
- How a data orchestration layer can simplify distributed data access and improve performance
- Real-world production use cases and example journeys for architecting a modern data platform
Driven by strong interests from our open-source community, the core team of Alluxio started to re-design an efficient and transparent way for users to leverage data orchestration through the POSIX interface. We have introduced a new JNI-based FUSE implementation to support POSIX data access, as well as many improvements in relevant data operations like more efficient distributedLoad, optimizations on listing or calculating directories with a massive amount of files, which are common in model training.
Today’s analytics workloads demand real-time access to expansive amounts of data. This session demonstrates how Alluxio’s data orchestration platform, running on Intel Optane persistent memory, accelerates access to this data and uncovers its valuable business insights faster.
RaptorX is an internal project name aiming to boost query latency significantly beyond what vanilla Presto is capable of. For this session, we introduce the hierarchical cache work including Alluxio data cache, fragment result cache, etc. Cache is the key building block for RaptorX. With the support of the cache, we are able to boost query performance by 10X. This new architecture can beat performance oriented connectors like Raptor with the added benefit of continuing to work with disaggregated storage.
Nowadays it is not straightforward to integrate Alluxio with popular query engines like Presto on existing Hive data. Solutions proposed by the community like Alluxio Catalog Service or Transparent URI brings unnecessary pressure on Alluxio masters when querying files should not be cached. This talk covers TikTok’s approach on adopting Alluxio for the cache layer without introducing additional services.
Alluxio has an excellent metrics system and supports various kinds of metrics, e.g. an embedded JSON sink and the prometheus sink. Users and developers can easily create a custom sink of Alluxio by implementing the Sink interface.
Also, Alluxio provides a metrics page in web UI to display some key information of Alluxio, such as bytes throughput and storage space. However, if you want a more flexible and universal monitoring, additional work is required.
Data Lake Analytics(DLA) is a large scale serverless data federation service on Alibaba Cloud. One of its serverless analytics engine is based on Presto. The DLA Presto engine supports a variety of data sources and is widely used in different application scenarios in the cloud. In this session, we will talk about the system architecture of DLA Presto engine, as well as the challenges and solutions. In particular, we will introduce the use of alluxio local cache to solve performance issues on OSS data sources caused by access delay and OSS bandwidth limitation. We will discuss the principle of alluxio local cache and some improvements we have made.