On-Demand Videos
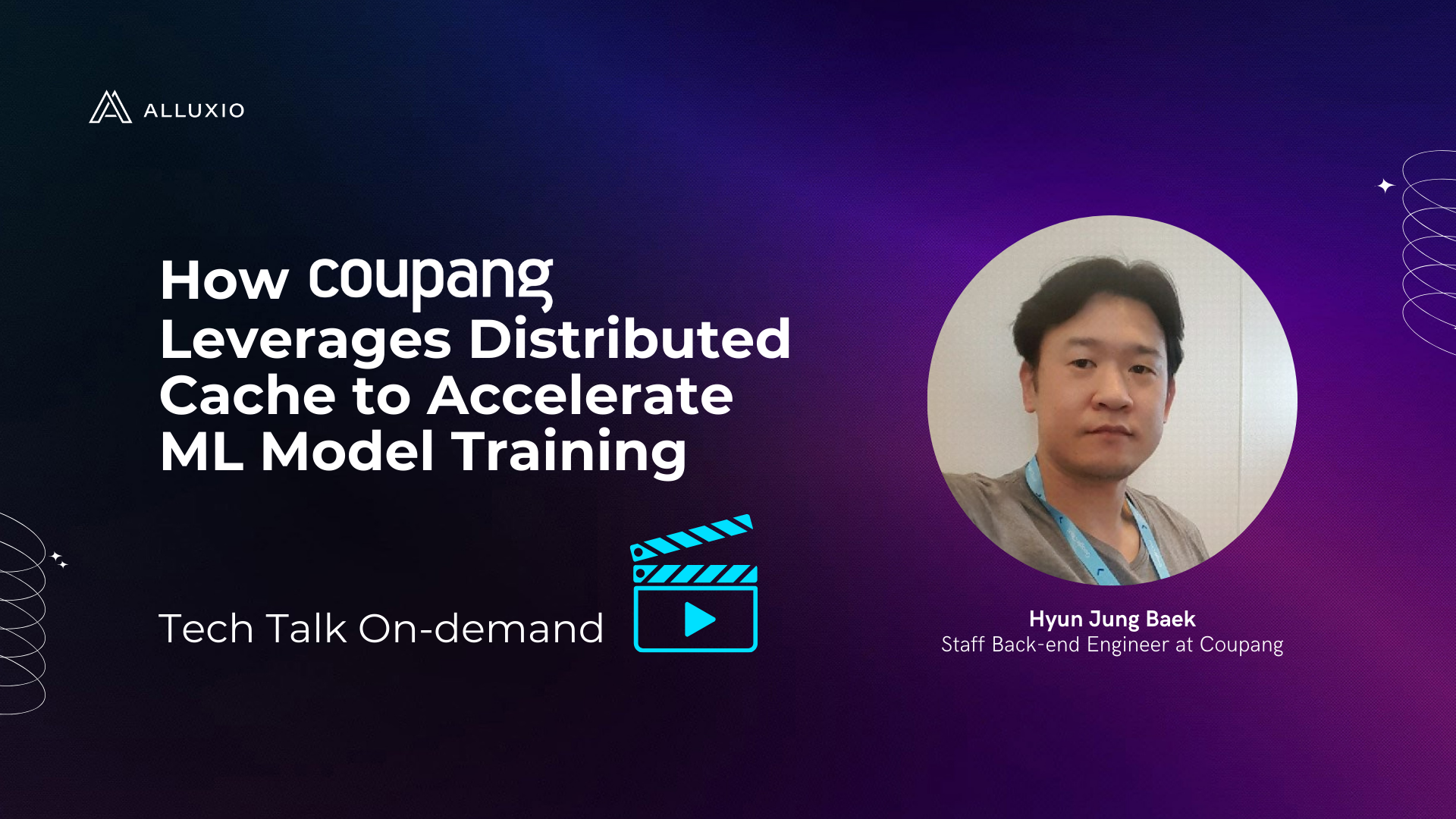
Coupang is a leading e-commerce company in South Korea, with over 50,000 employees and $20+ billion in annual revenue. Coupang's AI platform team builds and manages a large-scale AI platform in AWS for machine learning engineers to train models that enhance and customize product search results and product recommendations for its 100+ million customers.
As the search and recommendation models evolve, optimizing the underlying infrastructure for AI/ML workloads is essential for the e-commerce business. Coupang's platform team actively sought to improve their model training pipeline to boost machine learning engineers' productivity, publish models to production faster, and reduce operational costs.
Coupang focused on addressing several key areas:
- Shortening data preparation and model training time
- Improving GPU utilization in training clusters in different regions
- Reducing S3 API and egress costs incurred from copying large training datasets across regions
- Simplifying the operational complexity of storage system management
In this tech talk, Hyun Jung Baek, Staff Backend Engineer at Coupang, will share best practices for leveraging distributed caching to power search and recommendation model training infrastructure.
Hyun will discuss:
- How Coupang builds a world-class large-scale AI platform for machine learning engineers to deliver better search and recommendation models
- How adding distributed caching to their multi-region AI infrastructure improves GPU utilization, accelerates end-to-end training time, and significantly reduces cross-region data transfer costs.
- How to simplify platform operations and to easily deploy the same architecture to new GPU clusters.
About the Speaker
Hyun Jung Baek is a Staff Backend Engineer at Coupang.
Deepseek’s recent announcement of the Fire-flyer File System (3FS) has sparked excitement across the AI infra community, promising a breakthrough in how machine learning models access and process data.
In this webinar, an expert in distributed systems and AI infrastructure will take you inside Deepseek 3FS, the purpose-built file system for handling large files and high-bandwidth workloads. We’ll break down how 3FS optimizes data access and speeds up AI workloads as well as the design tradeoffs made to maximize throughput for AI workloads.
This webinar you’ll learn about how 3FS works under the hood, including:
✅ The system architecture
✅ Core software components
✅ Read/write flows
✅ Data distribution/placement algorithms
✅ Cluster/node management and disaster recovery
Whether you’re an AI researcher, ML engineer, or infrastructure architect, this deep dive will give you the technical insights you need to determine if 3FS is the right solution for you.
.png)
Data platform teams are increasingly challenged with accessing multiple data stores that are separated from compute engines, such as Spark, Presto, TensorFlow or PyTorch. Whether your data is distributed across multiple datacenters and/or clouds, a successful heterogeneous data platform requires efficient data access. Alluxio enables you to embrace the separation of storage from compute and use Alluxio data orchestration to simplify adoption of the data lake and data mesh paradigms for analytics and AI/ML workloads.
Join Alluxio’s Sr. Product Mgr., Adit Madan, to learn:
- Key challenges with architecting a successful heterogeneous data platform
- How data orchestration can overcome data access challenges in a distributed, heterogeneous environment
- How to identify ways to use Alluxio to meet the needs of your own data environment and workload requirements
ALLUXIO DAY IX 2022 January 21, 2022 Video: Presentation Slides: Industrial Bank's Alluxio Deployment from Alluxio, Inc.
ALLUXIO DAY IX 2022 January 21, 2022 Video: Presentation Slides: Vipshop Offline Data Cache Acceleration System – Alluxio Integration from Alluxio, Inc.
ALLUXIO DAY IX 2022 January 21, 2022 Video: Presentation Slides: The Evolution of an Open Data Platform with Alluxio from Alluxio, Inc.
Feifei Cai & Hao Zhu from WeRide provide an overview of Alluxio + Spark use case, which has been deployed and running in production to accelerate auto data tagging in the autonomous driving development.
This talk will introduce Apache Iceberg and its place in a modern and open data platform. It will cover the motivation for creating Iceberg at Netflix, as well as the data architecture that Iceberg makes possible.
This talk provides an overview of the read-after-write data consistent mechanism in the Alluxio system. Alluxio Core Maintainer and Presto Committer share their recent work on Alluxio and Apache Iceberg integration, as well as some recent work from the Presto community on Iceberg connector.
Apache Spark and Alluxio were both born in UC Berkeley’s AMPLab as research projects. As an open source data orchestration platform, Alluxio is able to achieve seamless docking and acceleration of different data sources, and improve the efficiency and fault tolerance of Spark’s big data computing business.
Alluxio has been deployed and running on a large scale managing petabytes level data in the production environment of companies such as Microsoft, Tiktok, Tencent, Singapore Development Bank, China Unicom, etc.
This talk shares the designs and use cases of the Alluxio and Spark integrated solutions, as well as the best practice and “what not to do” in designing and implementing Alluxio distributed systems.
In this talk, we will provide a complete picture of the Hudi platform components, along with their unique design choices. We will then deep dive into two important areas of active development going forward – table metadata management and caching. Specifically, we will discuss gaps in the data lake ecosystem around these aspects and provide strawman design approaches for Hudi aims to solve them going forward.
This talk discusses the opportunities and problems when Uber meets Alluxio. Zhongting from Uber will provide an overview of Uber traffic, cloud, distribution, invalidation, and consistent hashing. Beinan from Alluxio will provide a deep dive of metadata and monitoring metrics.
This talk describes the design of shadow cache, a lightweight component to track the working set size of Alluxio cache. Shadow cache can keep track of the working set size over the past window dynamically, and is implemented by a series of bloom filters. We’ve deployed the shadow cache in Facebook Presto and leverage the result to understand the system bottleneck and help with routing design decisions.
Alluxio’s capabilities as a Data Orchestration framework have encouraged users to onboard more of their data-driven applications to an Alluxio powered data access layer. Driven by strong interests from our open-source community, the core team of Alluxio started to re-design an efficient and transparent way for users to leverage data orchestration through the POSIX interface. This effort has a lot of progress with the collaboration with engineers from Microsoft, Alibaba and Tencent. Particularly, we have introduced a new JNI-based FUSE implementation to support POSIX data access, created a more efficient way to integrate Alluxio with FUSE service, as well as many improvements in relevant data operations like more efficient distributedLoad, optimizations on listing or calculating directories with a massive amount of files, which are common in model training. We will also share our engineering lessons and roadmap in future releases to support Machine Learning applications.