Prefill in LLM inference is known to be resource-intensive, especially for long LLM inputs. While better scheduling can mitigate prefill’s impact, it would be fundamentally better to avoid (most of) prefill. This talk introduces our preliminary effort towards drastically minimizing prefill delay for LLM inputs that naturally reuse text chunks, such as in retrieval-augmented generation. While keeping the KV cache of all text chunks in memory is difficult, we show that it is possible to store them on cheaper yet slower storage. By improving the loading process of the reused KV caches, we can still significantly speed up prefill delay while maintaining the same generation quality.
Video:
Presentation slides:
Videos:
Presentation Slides:
Complete the form below to access the full overview:
.png)
Videos
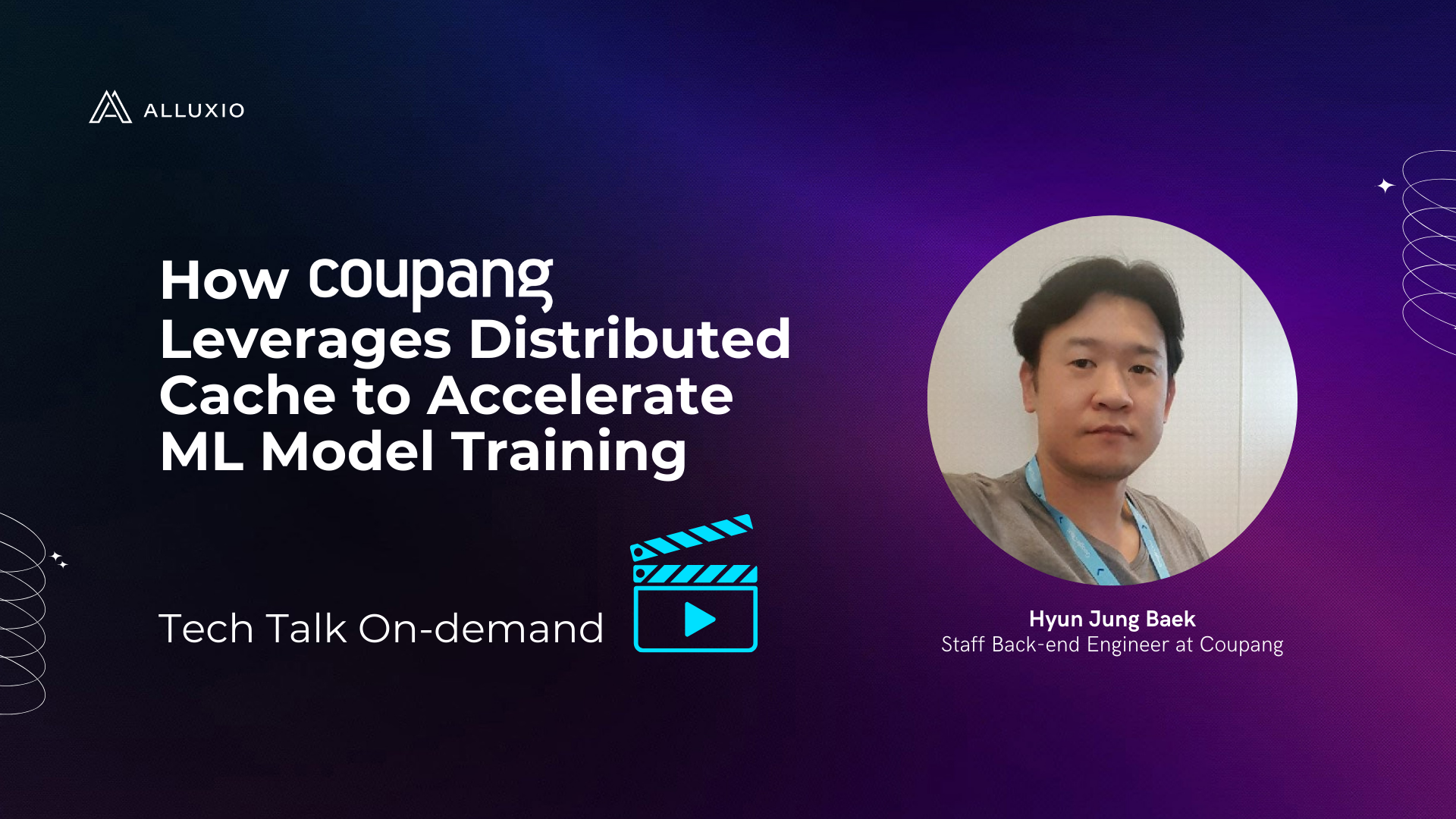
Coupang is a leading e-commerce company in South Korea, with over 50,000 employees and $20+ billion in annual revenue. Coupang's AI platform team builds and manages a large-scale AI platform in AWS for machine learning engineers to train models that enhance and customize product search results and product recommendations for its 100+ million customers.
As the search and recommendation models evolve, optimizing the underlying infrastructure for AI/ML workloads is essential for the e-commerce business. Coupang's platform team actively sought to improve their model training pipeline to boost machine learning engineers' productivity, publish models to production faster, and reduce operational costs.
Coupang focused on addressing several key areas:
- Shortening data preparation and model training time
- Improving GPU utilization in training clusters in different regions
- Reducing S3 API and egress costs incurred from copying large training datasets across regions
- Simplifying the operational complexity of storage system management
In this tech talk, Hyun Jung Baek, Staff Backend Engineer at Coupang, will share best practices for leveraging distributed caching to power search and recommendation model training infrastructure.
Hyun will discuss:
- How Coupang builds a world-class large-scale AI platform for machine learning engineers to deliver better search and recommendation models
- How adding distributed caching to their multi-region AI infrastructure improves GPU utilization, accelerates end-to-end training time, and significantly reduces cross-region data transfer costs.
- How to simplify platform operations and to easily deploy the same architecture to new GPU clusters.
About the Speaker
Hyun Jung Baek is a Staff Backend Engineer at Coupang.
Deepseek’s recent announcement of the Fire-flyer File System (3FS) has sparked excitement across the AI infra community, promising a breakthrough in how machine learning models access and process data.
In this webinar, an expert in distributed systems and AI infrastructure will take you inside Deepseek 3FS, the purpose-built file system for handling large files and high-bandwidth workloads. We’ll break down how 3FS optimizes data access and speeds up AI workloads as well as the design tradeoffs made to maximize throughput for AI workloads.
This webinar you’ll learn about how 3FS works under the hood, including:
✅ The system architecture
✅ Core software components
✅ Read/write flows
✅ Data distribution/placement algorithms
✅ Cluster/node management and disaster recovery
Whether you’re an AI researcher, ML engineer, or infrastructure architect, this deep dive will give you the technical insights you need to determine if 3FS is the right solution for you.