On-Demand Videos
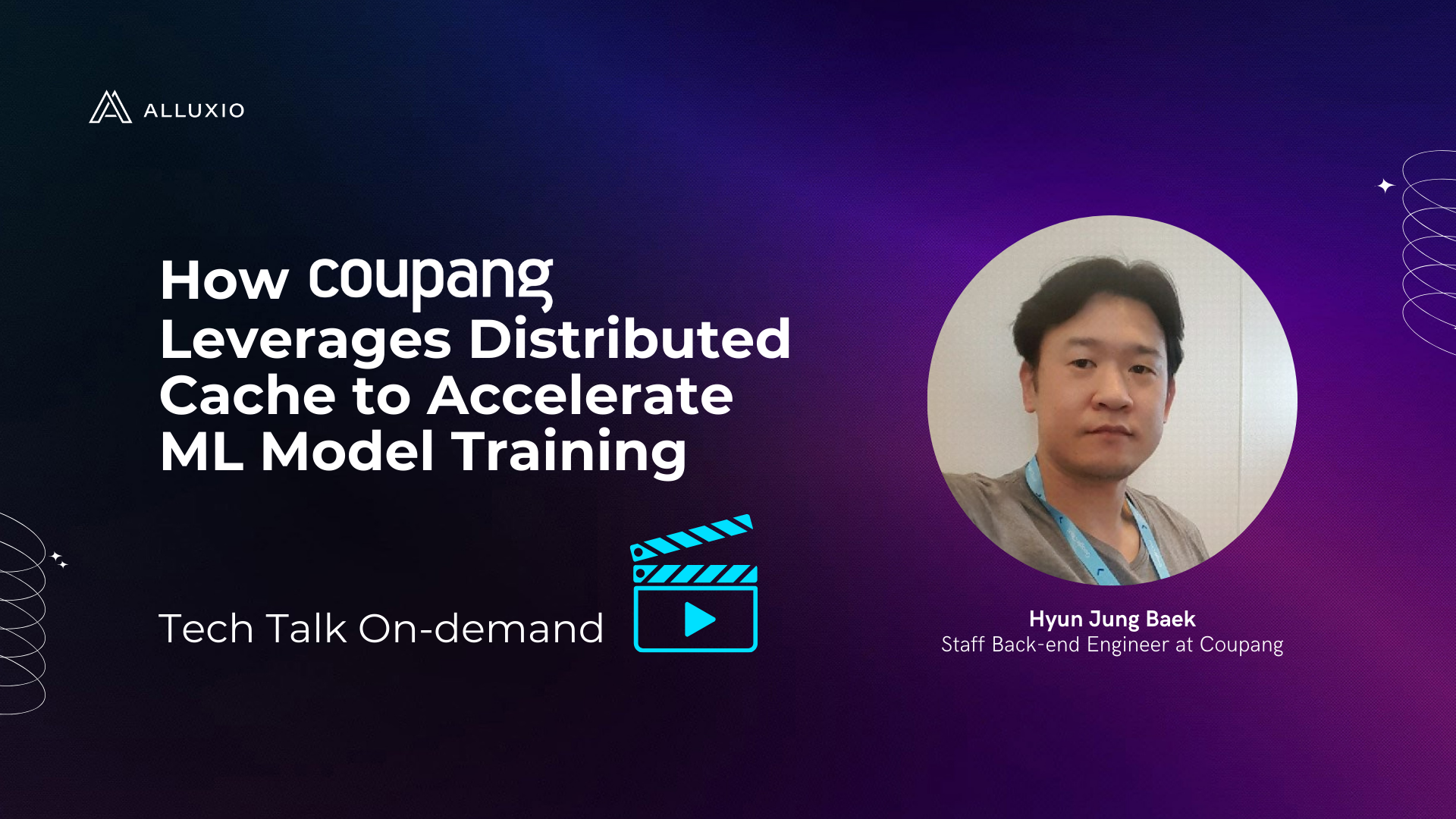
Coupang is a leading e-commerce company in South Korea, with over 50,000 employees and $20+ billion in annual revenue. Coupang's AI platform team builds and manages a large-scale AI platform in AWS for machine learning engineers to train models that enhance and customize product search results and product recommendations for its 100+ million customers.
As the search and recommendation models evolve, optimizing the underlying infrastructure for AI/ML workloads is essential for the e-commerce business. Coupang's platform team actively sought to improve their model training pipeline to boost machine learning engineers' productivity, publish models to production faster, and reduce operational costs.
Coupang focused on addressing several key areas:
- Shortening data preparation and model training time
- Improving GPU utilization in training clusters in different regions
- Reducing S3 API and egress costs incurred from copying large training datasets across regions
- Simplifying the operational complexity of storage system management
In this tech talk, Hyun Jung Baek, Staff Backend Engineer at Coupang, will share best practices for leveraging distributed caching to power search and recommendation model training infrastructure.
Hyun will discuss:
- How Coupang builds a world-class large-scale AI platform for machine learning engineers to deliver better search and recommendation models
- How adding distributed caching to their multi-region AI infrastructure improves GPU utilization, accelerates end-to-end training time, and significantly reduces cross-region data transfer costs.
- How to simplify platform operations and to easily deploy the same architecture to new GPU clusters.
About the Speaker
Hyun Jung Baek is a Staff Backend Engineer at Coupang.
Deepseek’s recent announcement of the Fire-flyer File System (3FS) has sparked excitement across the AI infra community, promising a breakthrough in how machine learning models access and process data.
In this webinar, an expert in distributed systems and AI infrastructure will take you inside Deepseek 3FS, the purpose-built file system for handling large files and high-bandwidth workloads. We’ll break down how 3FS optimizes data access and speeds up AI workloads as well as the design tradeoffs made to maximize throughput for AI workloads.
This webinar you’ll learn about how 3FS works under the hood, including:
✅ The system architecture
✅ Core software components
✅ Read/write flows
✅ Data distribution/placement algorithms
✅ Cluster/node management and disaster recovery
Whether you’re an AI researcher, ML engineer, or infrastructure architect, this deep dive will give you the technical insights you need to determine if 3FS is the right solution for you.
.png)
Machine learning models power Uber’s everyday business. However, developing and deploying a model is not a one-time event but a continuous process that requires careful planning, execution, and monitoring. In this session, Sally (Mihyong) Lee, Senior Staff Engineer & TLM @ Uber, highlights Uber’s practice on the machine learning lifecycle to ensure high model quality.
In this talk, Wanchao Liang, Software Engineer at Meta Pytorch Team, explores the technology advancements of PyTorch Distributed, and dives into the details of how multi-dimensional parallelism is made possible to train Large Language Models by composing different PyTorch native distributed training APIs.
ChatGPT and other massive models represents an amazing step forward in AI, yet they do not solve real-world business problems. In this session, Jordan Plawner, Global Director of Artificial Intelligence Product Manager and Strategy at Intel, surveys how the AI ecosystem has worked non-stop over this last year to take these all-purpose multi-task models and optimize them to they can be used by organizations to address domain specific problems. He explains these new AI-for-the-real world techniques and methods such as fine tuning and how they can be applied to deliver results which are highly performant with state-of-the-art accuracy while also being economical to build and deploy everywhere to enhance products and services.
This hands-on session discusses best practices for using PyTorch and Alluxio during model training on AWS. Shawn and Lu provide a step-by-step demonstration of how to use Alluxio on EKS as a distributed cache to accelerate computer vision model training jobs that read datasets from S3. This architecture significantly improves the utilization of GPUs from 30% to 90%+, archives ~5x faster training, and lower cloud storage costs.
As enterprises race to roll out artificial intelligence, often overlookModel training requires extensive computational and GPU resources. When training models on AWS, loading data from S3 often becomes a major bottleneck, wasting valuable GPU cycles. Optimizing data loading can greatly reduce GPU idle time and increase GPU utilization.
In this webinar, Greg Palmer will discuss best practices for efficient data loading during model training on AWS. He will demonstrate how to use Alluxio on EKS as a distributed cache to accelerate PyTorch training jobs that read datasets from S3. This architecture significantly improves the utilization of GPUs from 30% to 90%+, archives ~5x faster training, and lower cloud storage costs.
What you will learn:
- The challenges of feeding data-hungry GPUs in the cloud
- How to accelerate model training by optimizing data loading on AWS
- The reference architecture for running PyTorch jobs with Alluxio cache on EKS while reading data from S3, with benchmark results of training ResNet50 and BERT
- How to use TensorBoard to identify bottlenecks in GPU utilization
As enterprises race to roll out artificial intelligence, often overlooked are the infrastructure needs to support scalable ML model development and deployment. Efforts to effectively access and utilize GPUs often lead to extensive data engineering managing data copies or specialized storage, leading to out-of-control cloud and infrastructure costs.
To address the challenges, enterprises need a new data access layer to connect compute engines to data stores wherever they reside in distributed environments.
Join this webinar with Kevin Petrie, Eckerson Group VP of Research, and Sridhar Venkatesh, Alluxio SVP of Product, to explore tools, techniques, and best practices to remove data access bottlenecks and accelerate AI/ML model training. You will learn:
- Modern requirements for AI/ML model training and data engineering
- The challenges of GPU utilization in machine learning and the need for specialized hardware
- How a new data access layer connects compute to data stores across environments
- Best practices for optimizing ML training and guiding principles for success
Organizations are retooling their enterprise data infrastructure in the race for AI/ML. However, growing datasets, extensive data engineering overhead, high GPU costs, and expensive specialized storage can make it difficult to get fast results from model development.
The data access layer is the key to accelerating your path to AI/ML. In this webinar, Roland Theron, Senior Solutions Engineer at Alluxio, discusses how the data access layer can help you:
- Build AI architecture on your existing data lake without the need for specialized hardware.
- Streamline the time-consuming process of managing data copies in data engineering.
- Speed up training workloads with high GPU utilization.
- Achieve optimal concurrency to deliver models to inference clusters for demanding applications
Join us with David Loshin, President of Knowledge Integrity, and Sridhar Venkatesh, SVP of Product at Alluxio, to learn more about the infrastructure hurdles associated with AI/ML model training and deployment and how to overcome them. Topics include:
- The challenges of AI and model training
- GPU utilization in machine learning and the need for specialized hardware
- Managing data access and maintaining a source of truth in data lakes
- Best practices for optimizing ML training
When training models on ultra-large datasets, one of the biggest challenges is low GPU utilization. These powerful processors are often underutilized due to inefficient I/O and data access. This mismatch between computation and storage leads to wasted GPU resources, low performance, and high cloud storage costs. The rise of generative AI and GPU scarcity is only making this problem worse.
In this webinar, Tarik and Beinan discuss strategies for transforming idle GPUs into optimal powerhouses. They will focus on cost-effective management of ultra-large datasets for AI and analytics.
What you will learn:
- The challenges of I/O stalls leading to low GPU utilization for model training
- High-performance, high-throughput data access (I/O) strategies
- The benefits of using an on-demand data access layer over your storage
- How Uber addresses managing ultra-large datasets using high-density storage and caching
As the AI landscape rapidly evolves, the advancements in generative AI technologies, such as ChatGPT, are driving a need for robust data infrastructures tailored for large language model (LLM) training and inference in the cloud. To effectively leverage the breakthroughs in LLM, organizations must ensure low latency, high concurrency, and scalability in production environments.
In this Alluxio-hosted webinar, Shouwei presented on the design and implementation of a distributed caching system that addresses the I/O challenges of LLM training and inference. He explored the unique requirements of data access patterns and offer practical best practices for optimizing the data pipeline through distributed caching in the cloud. The session featured insights from real-world examples, such as Microsoft, Tencent, and Zhihu, as well as from the open-source community. Watch this recording to get a deeper understanding of how to harness scalable, efficient, and robust data infrastructures for LLM training and inference.