ALLUXIO DAY XV 2022
September 15, 2022
Streaming systems form the backbone of the modern data pipeline as the stream processing capabilities provide insights on events as they arrive. But what if we want to go further than this and execute analytical queries on this real-time data? That’s where Apache Pinot comes in.
OLAP databases used for analytical workloads traditionally executed queries on yesterday’s data with query latency in the 10s of seconds. The emergence of real-time analytics has changed all this and the expectation is that we should now be able to run thousands of queries per second on fresh data with query latencies typically seen on OLTP databases.
Apache Pinot is a realtime distributed OLAP datastore, which is used to deliver scalable real time analytics with low latency. It can ingest data from streaming sources like Kafka, as well as from batch data sources (S3, HDFS, Azure Data Lake, Google Cloud Storage), and provides a layer of indexing techniques that can be used to maximize the performance of queries.
Come to this talk to learn how you can add real-time analytics capability to your data pipeline.
ALLUXIO DAY XV 2022
September 15, 2022
Streaming systems form the backbone of the modern data pipeline as the stream processing capabilities provide insights on events as they arrive. But what if we want to go further than this and execute analytical queries on this real-time data? That’s where Apache Pinot comes in.
OLAP databases used for analytical workloads traditionally executed queries on yesterday’s data with query latency in the 10s of seconds. The emergence of real-time analytics has changed all this and the expectation is that we should now be able to run thousands of queries per second on fresh data with query latencies typically seen on OLTP databases.
Apache Pinot is a realtime distributed OLAP datastore, which is used to deliver scalable real time analytics with low latency. It can ingest data from streaming sources like Kafka, as well as from batch data sources (S3, HDFS, Azure Data Lake, Google Cloud Storage), and provides a layer of indexing techniques that can be used to maximize the performance of queries.
Come to this talk to learn how you can add real-time analytics capability to your data pipeline.
Video:
Presentation Slides:
Videos:
Presentation Slides:
Complete the form below to access the full overview:
.png)
Videos
Nilesh Agarwal, Co-founder & CTO at Inferless, shares insights on accelerating LLM inference in the cloud using Alluxio, tackling key bottlenecks like slow model weight loading from S3 and lengthy container startup time. Inferless uses Alluxio as a three-tier cache system that dramatically cuts model load time by 10x.
In this talk, Jingwen Ouyang, Senior Product Manager at Alluxio, will share how Alluxio make it easy to share and manage data from any storage to any compute engine in any environment with high performance and low cost for your model training, model inference, and model distribution workload.
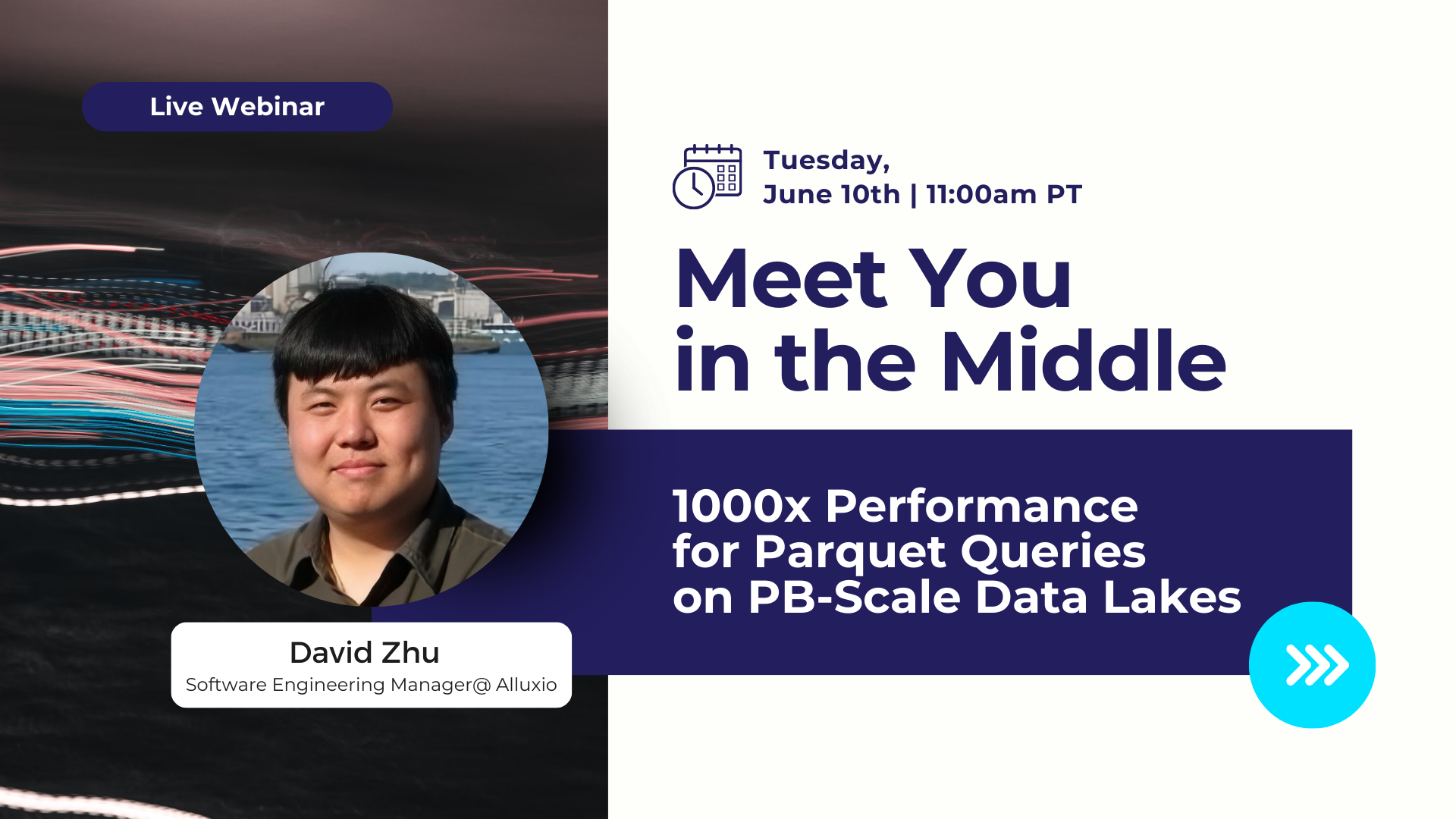
Storing data as Parquet files on cloud object storage, such as AWS S3, has become prevalent not only for large-scale data lakes but also as lightweight feature stores for training and inference, or as document stores for Retrieval-Augmented Generation (RAG). However, querying petabyte-to-exabyte-scale data lakes directly from S3 remains notoriously slow, with latencies typically ranging from hundreds of milliseconds to several seconds.
In this webinar, David Zhu, Software Engineering Manager at Alluxio, will present the results of a joint collaboration between Alluxio and a leading SaaS and data infrastructure enterprise that explored leveraging Alluxio as a high-performance caching and acceleration layer atop AWS S3 for ultra-fast querying of Parquet files at PB scale.
David will share:
- How Alluxio delivers sub-millisecond Time-to-First-Byte (TTFB) for Parquet queries, comparable to S3 Express One Zone, without requiring specialized hardware, data format changes, or data migration from your existing data lake.
- The architecture that enables Alluxio’s throughput to scale linearly with cluster size, achieving one million queries per second on a modest 50-node deployment, surpassing S3 Express single-account throughput by 50x without latency degradation.
- Specifics on how Alluxio offloads partial Parquet read operations and reduces overhead, enabling direct, ultra-low-latency point queries in hundreds of microseconds and achieving a 1,000x performance gain over traditional S3 querying methods.
Speaker: David Zhu
David Zhu is a Software Engineer Manager at Alluxio. At Alluxio, David focuses on metadata management and end-to-end performance benchmarking and optimizations. Prior to that, David completed his Ph.D. from UC Berkeley, with a focus on distributed data management systems and operating systems for the data center. David also holds a Bachelor of Software Engineering from the University of Waterloo.