Big Data Bellevue Meetup
May 19, 2022
Today, data engineering in modern enterprises has become increasingly more complex and resource-consuming, particularly because (1) the rich amount of organizational data is often distributed across data centers, cloud regions, or even cloud providers, and (2) the complexity of the big data stack has been quickly increasing over the past few years with an explosion in big-data analytics and machine-learning engines (like MapReduce, Hive, Spark, Presto, Tensorflow, PyTorch to name a few).
To address these challenges, it is critical to provide a single and logical namespace to federate different storage services, on-prem or cloud-native, to abstract away the data heterogeneity, while providing data locality to improve the computation performance. [Bin Fan] will share his observation and lessons learned in designing, architecting, and implementing such a system – Alluxio open-source project — since 2015.
Alluxio originated from UC Berkeley AMPLab (used to be called Tachyon) and was initially proposed as a daemon service to enable Spark to share RDDs across jobs for performance and fault tolerance. Today, it has become a general-purpose, high-performance, and highly available distributed file system to provide generic data service to abstract away complexity in data and I/O. Many companies and organizations today like Uber, Meta, Tencent, Tiktok, Shopee are using Alluxio in production, as a building block in their data platform to create a data abstraction and access layer. We will talk about the journey of this open source project, especially in its design challenges in tiered metadata storage (based on RocksDB), embedded state-replicate machine (based on RAFT) for HA, and evolution in RPC framework (based on gRPC) and etc.
Meetup Group
Big Data Bellevue: https://www.meetup.com/big-data-bellevue-bdb/
Big Data Bellevue Meetup
May 19, 2022
Today, data engineering in modern enterprises has become increasingly more complex and resource-consuming, particularly because (1) the rich amount of organizational data is often distributed across data centers, cloud regions, or even cloud providers, and (2) the complexity of the big data stack has been quickly increasing over the past few years with an explosion in big-data analytics and machine-learning engines (like MapReduce, Hive, Spark, Presto, Tensorflow, PyTorch to name a few).
To address these challenges, it is critical to provide a single and logical namespace to federate different storage services, on-prem or cloud-native, to abstract away the data heterogeneity, while providing data locality to improve the computation performance. [Bin Fan] will share his observation and lessons learned in designing, architecting, and implementing such a system – Alluxio open-source project — since 2015.
Alluxio originated from UC Berkeley AMPLab (used to be called Tachyon) and was initially proposed as a daemon service to enable Spark to share RDDs across jobs for performance and fault tolerance. Today, it has become a general-purpose, high-performance, and highly available distributed file system to provide generic data service to abstract away complexity in data and I/O. Many companies and organizations today like Uber, Meta, Tencent, Tiktok, Shopee are using Alluxio in production, as a building block in their data platform to create a data abstraction and access layer. We will talk about the journey of this open source project, especially in its design challenges in tiered metadata storage (based on RocksDB), embedded state-replicate machine (based on RAFT) for HA, and evolution in RPC framework (based on gRPC) and etc.
Meetup Group
Big Data Bellevue: https://www.meetup.com/big-data-bellevue-bdb/
Big Data Bellevue Meetup
May 19, 2022
Today, data engineering in modern enterprises has become increasingly more complex and resource-consuming, particularly because (1) the rich amount of organizational data is often distributed across data centers, cloud regions, or even cloud providers, and (2) the complexity of the big data stack has been quickly increasing over the past few years with an explosion in big-data analytics and machine-learning engines (like MapReduce, Hive, Spark, Presto, Tensorflow, PyTorch to name a few).
To address these challenges, it is critical to provide a single and logical namespace to federate different storage services, on-prem or cloud-native, to abstract away the data heterogeneity, while providing data locality to improve the computation performance. [Bin Fan] will share his observation and lessons learned in designing, architecting, and implementing such a system – Alluxio open-source project — since 2015.
Alluxio originated from UC Berkeley AMPLab (used to be called Tachyon) and was initially proposed as a daemon service to enable Spark to share RDDs across jobs for performance and fault tolerance. Today, it has become a general-purpose, high-performance, and highly available distributed file system to provide generic data service to abstract away complexity in data and I/O. Many companies and organizations today like Uber, Meta, Tencent, Tiktok, Shopee are using Alluxio in production, as a building block in their data platform to create a data abstraction and access layer. We will talk about the journey of this open source project, especially in its design challenges in tiered metadata storage (based on RocksDB), embedded state-replicate machine (based on RAFT) for HA, and evolution in RPC framework (based on gRPC) and etc.
Meetup Group
Big Data Bellevue: https://www.meetup.com/big-data-bellevue-bdb/
Video:
Presentation Slides:
Videos:
Presentation Slides:
Complete the form below to access the full overview:
.png)
Videos
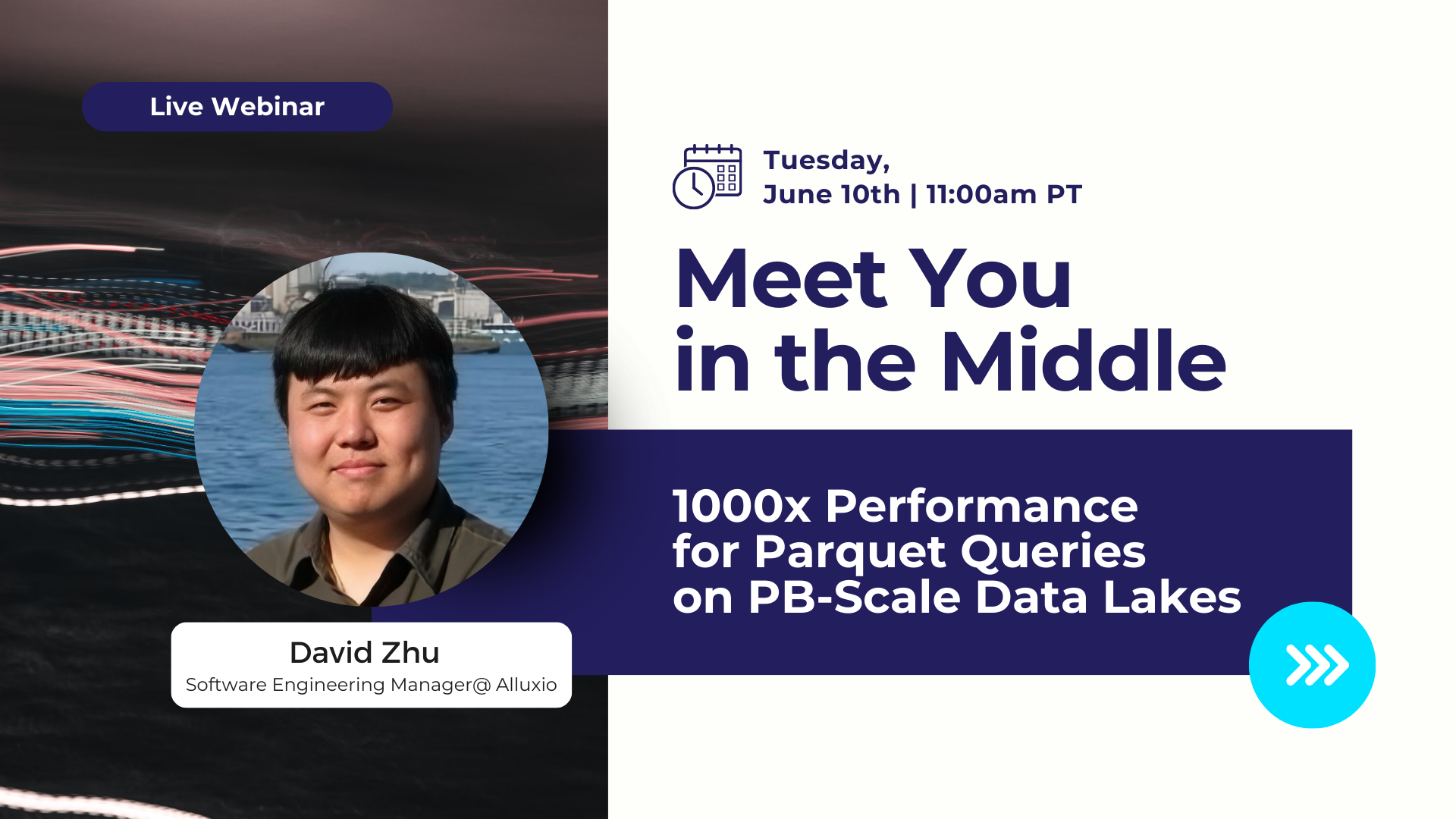
Storing data as Parquet files on cloud object storage, such as AWS S3, has become prevalent not only for large-scale data lakes but also as lightweight feature stores for training and inference, or as document stores for Retrieval-Augmented Generation (RAG). However, querying petabyte-to-exabyte-scale data lakes directly from S3 remains notoriously slow, with latencies typically ranging from hundreds of milliseconds to several seconds.
In this webinar, David Zhu, Software Engineering Manager at Alluxio, will present the results of a joint collaboration between Alluxio and a leading SaaS and data infrastructure enterprise that explored leveraging Alluxio as a high-performance caching and acceleration layer atop AWS S3 for ultra-fast querying of Parquet files at PB scale.
David will share:
- How Alluxio delivers sub-millisecond Time-to-First-Byte (TTFB) for Parquet queries, comparable to S3 Express One Zone, without requiring specialized hardware, data format changes, or data migration from your existing data lake.
- The architecture that enables Alluxio’s throughput to scale linearly with cluster size, achieving one million queries per second on a modest 50-node deployment, surpassing S3 Express single-account throughput by 50x without latency degradation.
- Specifics on how Alluxio offloads partial Parquet read operations and reduces overhead, enabling direct, ultra-low-latency point queries in hundreds of microseconds and achieving a 1,000x performance gain over traditional S3 querying methods.
Speaker: David Zhu
David Zhu is a Software Engineer Manager at Alluxio. At Alluxio, David focuses on metadata management and end-to-end performance benchmarking and optimizations. Prior to that, David completed his Ph.D. from UC Berkeley, with a focus on distributed data management systems and operating systems for the data center. David also holds a Bachelor of Software Engineering from the University of Waterloo.

Coupang is a leading e-commerce company in South Korea, with over 50,000 employees and $20+ billion in annual revenue. Coupang's AI platform team builds and manages a large-scale AI platform in AWS for machine learning engineers to train models that enhance and customize product search results and product recommendations for its 100+ million customers.
As the search and recommendation models evolve, optimizing the underlying infrastructure for AI/ML workloads is essential for the e-commerce business. Coupang's platform team actively sought to improve their model training pipeline to boost machine learning engineers' productivity, publish models to production faster, and reduce operational costs.
Coupang focused on addressing several key areas:
- Shortening data preparation and model training time
- Improving GPU utilization in training clusters in different regions
- Reducing S3 API and egress costs incurred from copying large training datasets across regions
- Simplifying the operational complexity of storage system management
In this tech talk, Hyun Jung Baek, Staff Backend Engineer at Coupang, will share best practices for leveraging distributed caching to power search and recommendation model training infrastructure.
Hyun will discuss:
- How Coupang builds a world-class large-scale AI platform for machine learning engineers to deliver better search and recommendation models
- How adding distributed caching to their multi-region AI infrastructure improves GPU utilization, accelerates end-to-end training time, and significantly reduces cross-region data transfer costs.
- How to simplify platform operations and to easily deploy the same architecture to new GPU clusters.
About the Speaker
Hyun Jung Baek is a Staff Backend Engineer at Coupang.
This video is originally published on TechArena.
At NVIDIA GTC 2025, Bin Fan from Alluxio and Scott Shadley from Solidigm tackled the growing need for decoupled storage and compute in AI infrastructure. They explained how Alluxio's caching layer enables fast, scalable and reliable infrastructure, accelerating AI training and inferencing seamlessly across regions and platforms.