What’s Presto Summit? It’s the leading Presto conference co-organized by our partner Starburst Data and the Presto Software Foundation.
Overview of the Summit
- Presto is among the fastest growing open source analytical query frameworks with production use cases across industries such as retail, telco, tech and more
- This was a full house event at Twitter HQ with more than 150 attendees
- Excellent keynote on the future of Presto delivered by Martin Traverso, Dain Sundstrom, and David Phillips – the co-creators of Presto and co-founders of the Presto Software Foundation (slides)
What We Learned
- Presto is high performance and has rich functionalities designed for interactive SQL queries
- Rise of cloud deployments of Presto both all cloud as well as hybrid cloud are growing. There is a need to simplify hybrid deployments which currently include making copies of data in the cloud and in some cases to HDFS and managing the Hadoop cluster.
- Big data workloads are increasingly looking for simpler compute orchestration and adopting Kubernetes. Starburst Presto announced a new Kubernetes operator to simplify deployment and scaling of clusters.
Reasons to try the Presto, Alluxio, and Any Storage Stack
- High query performance without operational overhead of data copying or ETL
- Query on data anywhere: hybrid, public cloud, on-premise
- Consistent and low latency
Learn more: Starburst Presto and Alluxio announce strategic OEM partnership | Presto with Alluxio | Download Alluxio
Additional resources:
- Community office hour (virtual): Building Fast SQL Analytics with Presto, Alluxio, and S3
- Got questions? Chat with Alluxio experts on Slack
.png)
Blog
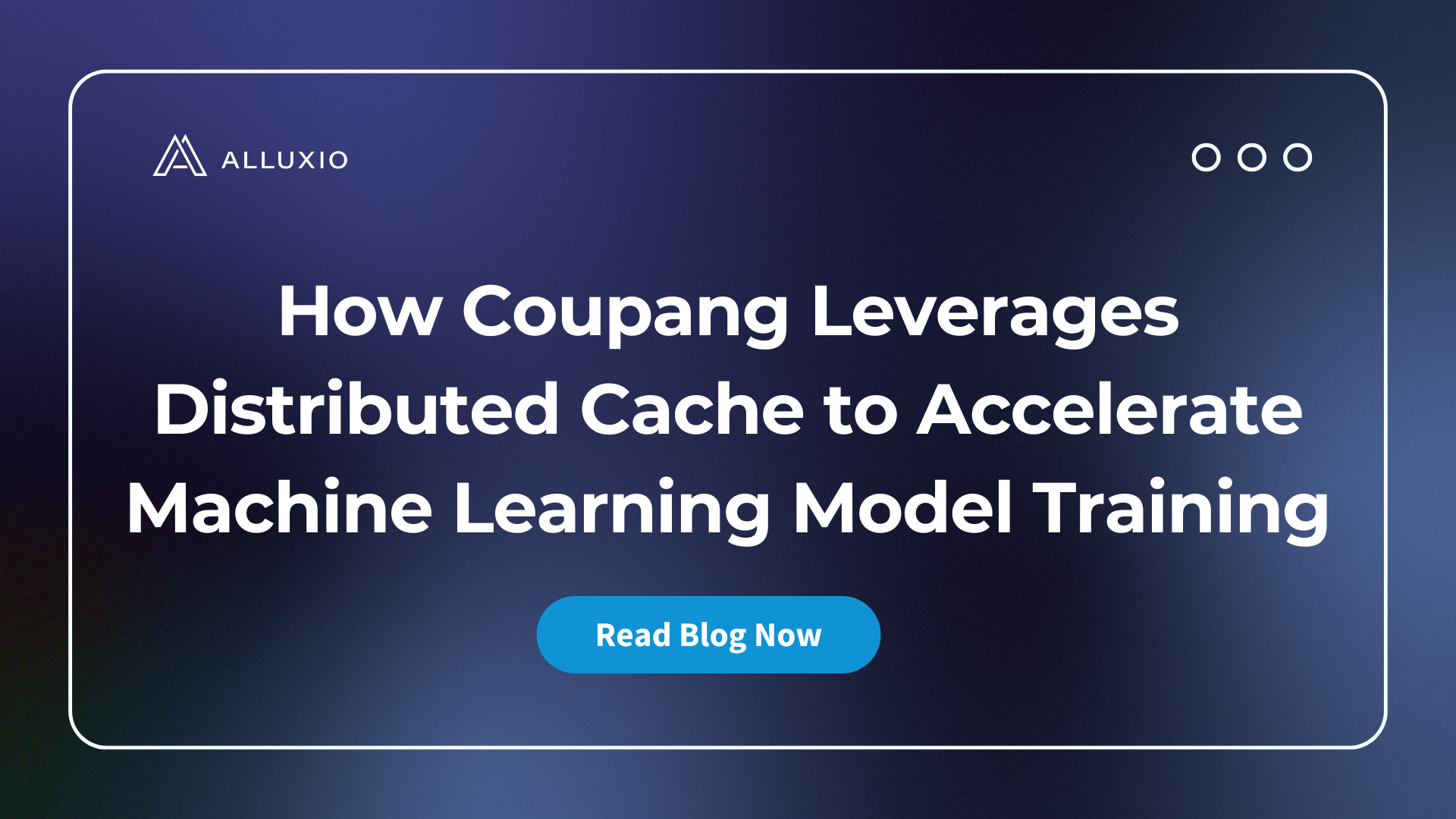
Coupang, a Fortune 200 technology company, manages a multi-cluster GPU architecture for their AI/ML model training. This architecture introduced significant challenges, including:
- Time-consuming data preparation and data copy/movement
- Difficulty utilizing GPU resources efficiently
- High and growing storage costs
- Excessive operational overhead maintaining storage for localized data silos
To resolve these challenges, Coupang’s AI platform team implemented a distributed caching system that automatically retrieves training data from their central data lake, improves data loading performance, unifies access paths for model developers, automates data lifecycle management, and extends easily across Kubernetes environments. The new distributed caching architecture has improved model training speed, reduced storage costs, increased GPU utilization across clusters, lowered operational overhead, enabled training workload portability, and delivered 40% better I/O performance compared to parallel file systems.
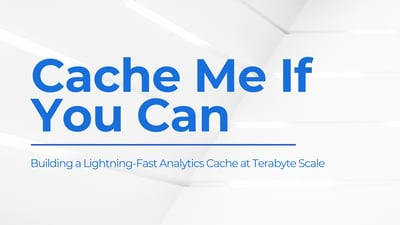
Suresh Kumar Veerapathiran and Anudeep Kumar, engineering leaders at Uptycs, recently shared their experience of evolving their data platform and analytics architecture to power analytics through a generative AI interface. In their post on Medium titled Cache Me If You Can: Building a Lightning-Fast Analytics Cache at Terabyte Scale, Veerapathiran and Kumar provide detailed insights into the challenges they faced (and how they solved them) scaling their analytics solution that collects and reports on terabytes of telemetry data per day as part of Uptycs Cloud-Native Application Protection Platform (CNAPP) solutions.