Alluxio foresaw the need for agility when accessing data across silos separated from compute engines like Spark, Presto, Tensorflow and PyTorch. Embracing the separation of storage from compute, the Alluxio data orchestration platform simplifies adoption of the data lake and data mesh paradigm for analytics and AI/ML. In this talk, Bin Fan will share observations to help identify ways to use the platform to meet the needs of your data environment and workloads.
越來越多的企業架構已轉向混合雲和多雲環境。雖然這種轉變帶來了更大的靈活性和敏捷性,但也意味著必須將計算與存儲分離,這就對企業跨框架、跨雲和跨存儲系統的數據管理和編排提出了新的挑戰。此分享將讓聽眾深入了解Alluxio數據編排理念在數據中台對存儲和計算的解耦作用,以及數據編排針對存算分離場景提出的創新架構,同時結合來自金融、運營商、互聯網等行業的典型應用場景來展現Alluxio如何為大數據計算帶來真正的加速,以及如何將數據編排技術用於AI模型訓練!
*This is a bilingual presentation.
Alluxio foresaw the need for agility when accessing data across silos separated from compute engines like Spark, Presto, Tensorflow and PyTorch. Embracing the separation of storage from compute, the Alluxio data orchestration platform simplifies adoption of the data lake and data mesh paradigm for analytics and AI/ML. In this talk, Bin Fan will share observations to help identify ways to use the platform to meet the needs of your data environment and workloads.
越來越多的企業架構已轉向混合雲和多雲環境。雖然這種轉變帶來了更大的靈活性和敏捷性,但也意味著必須將計算與存儲分離,這就對企業跨框架、跨雲和跨存儲系統的數據管理和編排提出了新的挑戰。此分享將讓聽眾深入了解Alluxio數據編排理念在數據中台對存儲和計算的解耦作用,以及數據編排針對存算分離場景提出的創新架構,同時結合來自金融、運營商、互聯網等行業的典型應用場景來展現Alluxio如何為大數據計算帶來真正的加速,以及如何將數據編排技術用於AI模型訓練!
*This is a bilingual presentation.
Alluxio foresaw the need for agility when accessing data across silos separated from compute engines like Spark, Presto, Tensorflow and PyTorch. Embracing the separation of storage from compute, the Alluxio data orchestration platform simplifies adoption of the data lake and data mesh paradigm for analytics and AI/ML. In this talk, Bin Fan will share observations to help identify ways to use the platform to meet the needs of your data environment and workloads.
越來越多的企業架構已轉向混合雲和多雲環境。雖然這種轉變帶來了更大的靈活性和敏捷性,但也意味著必須將計算與存儲分離,這就對企業跨框架、跨雲和跨存儲系統的數據管理和編排提出了新的挑戰。此分享將讓聽眾深入了解Alluxio數據編排理念在數據中台對存儲和計算的解耦作用,以及數據編排針對存算分離場景提出的創新架構,同時結合來自金融、運營商、互聯網等行業的典型應用場景來展現Alluxio如何為大數據計算帶來真正的加速,以及如何將數據編排技術用於AI模型訓練!
*This is a bilingual presentation.
Videos:
Presentation Slides:
Complete the form below to access the full overview:
.png)
Videos
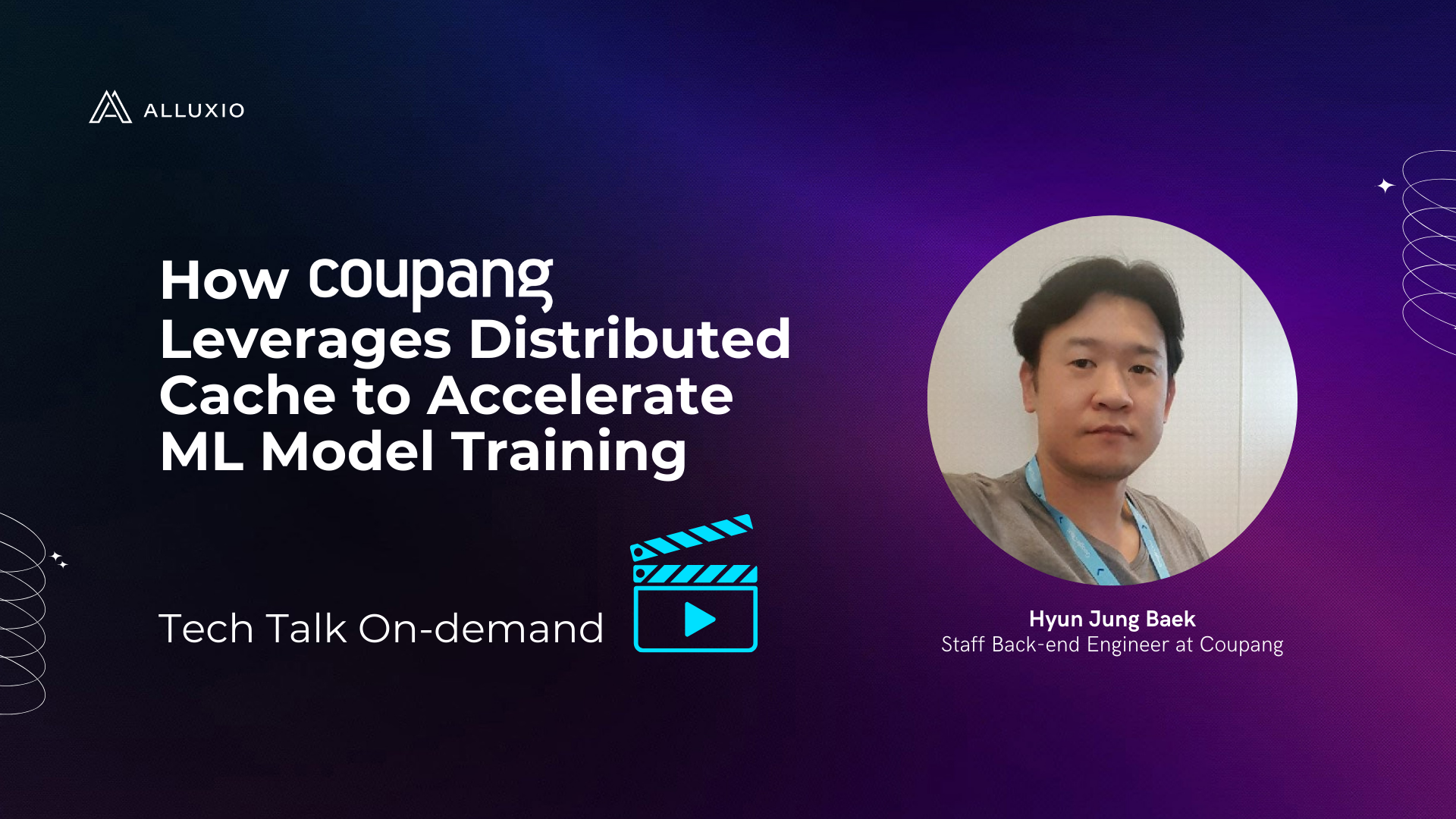
Coupang is a leading e-commerce company in South Korea, with over 50,000 employees and $20+ billion in annual revenue. Coupang's AI platform team builds and manages a large-scale AI platform in AWS for machine learning engineers to train models that enhance and customize product search results and product recommendations for its 100+ million customers.
As the search and recommendation models evolve, optimizing the underlying infrastructure for AI/ML workloads is essential for the e-commerce business. Coupang's platform team actively sought to improve their model training pipeline to boost machine learning engineers' productivity, publish models to production faster, and reduce operational costs.
Coupang focused on addressing several key areas:
- Shortening data preparation and model training time
- Improving GPU utilization in training clusters in different regions
- Reducing S3 API and egress costs incurred from copying large training datasets across regions
- Simplifying the operational complexity of storage system management
In this tech talk, Hyun Jung Baek, Staff Backend Engineer at Coupang, will share best practices for leveraging distributed caching to power search and recommendation model training infrastructure.
Hyun will discuss:
- How Coupang builds a world-class large-scale AI platform for machine learning engineers to deliver better search and recommendation models
- How adding distributed caching to their multi-region AI infrastructure improves GPU utilization, accelerates end-to-end training time, and significantly reduces cross-region data transfer costs.
- How to simplify platform operations and to easily deploy the same architecture to new GPU clusters.
About the Speaker
Hyun Jung Baek is a Staff Backend Engineer at Coupang.
Deepseek’s recent announcement of the Fire-flyer File System (3FS) has sparked excitement across the AI infra community, promising a breakthrough in how machine learning models access and process data.
In this webinar, an expert in distributed systems and AI infrastructure will take you inside Deepseek 3FS, the purpose-built file system for handling large files and high-bandwidth workloads. We’ll break down how 3FS optimizes data access and speeds up AI workloads as well as the design tradeoffs made to maximize throughput for AI workloads.
This webinar you’ll learn about how 3FS works under the hood, including:
✅ The system architecture
✅ Core software components
✅ Read/write flows
✅ Data distribution/placement algorithms
✅ Cluster/node management and disaster recovery
Whether you’re an AI researcher, ML engineer, or infrastructure architect, this deep dive will give you the technical insights you need to determine if 3FS is the right solution for you.