Blog
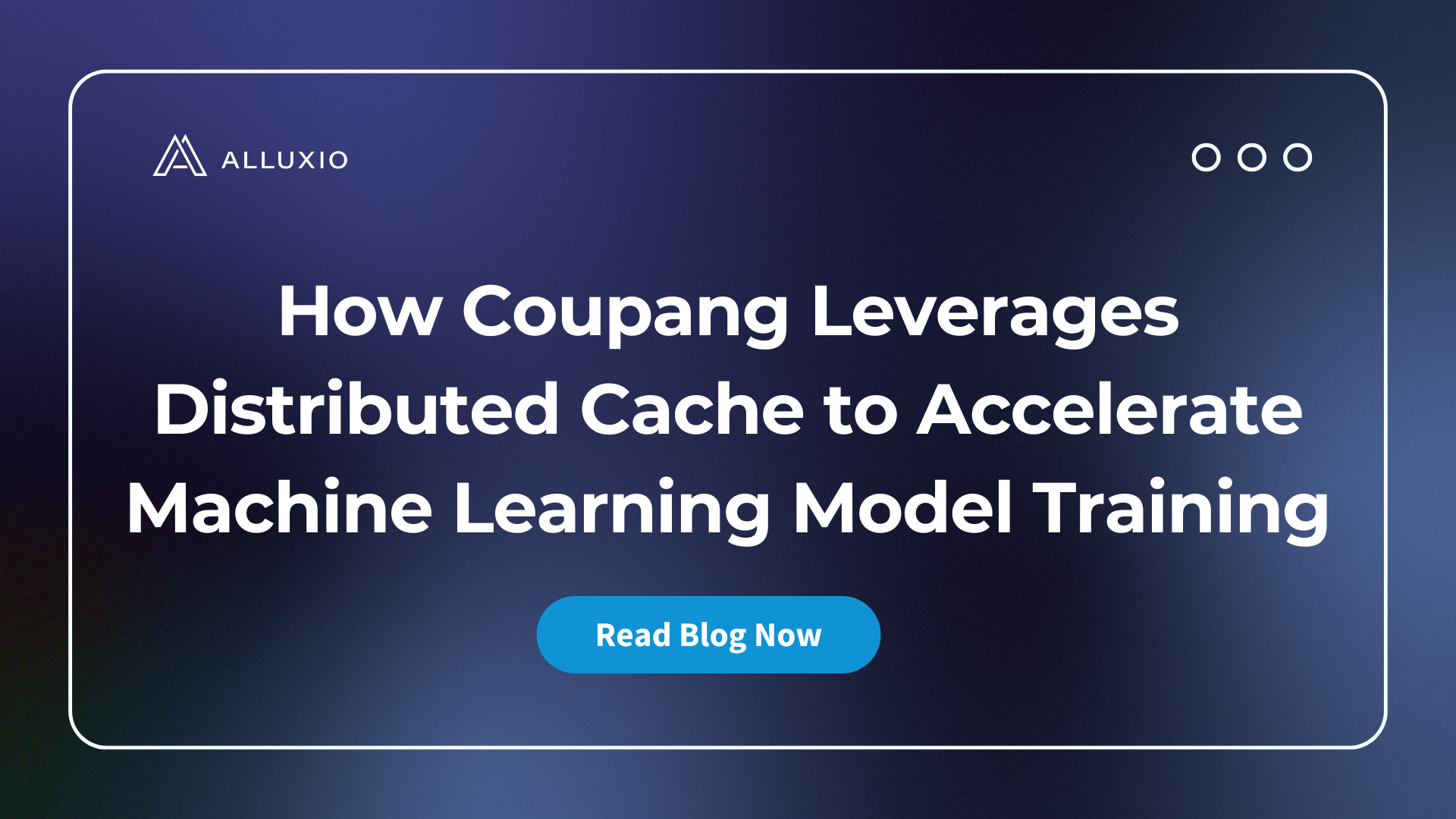
Coupang, a Fortune 200 technology company, manages a multi-cluster GPU architecture for their AI/ML model training. This architecture introduced significant challenges, including:
- Time-consuming data preparation and data copy/movement
- Difficulty utilizing GPU resources efficiently
- High and growing storage costs
- Excessive operational overhead maintaining storage for localized data silos
To resolve these challenges, Coupang’s AI platform team implemented a distributed caching system that automatically retrieves training data from their central data lake, improves data loading performance, unifies access paths for model developers, automates data lifecycle management, and extends easily across Kubernetes environments. The new distributed caching architecture has improved model training speed, reduced storage costs, increased GPU utilization across clusters, lowered operational overhead, enabled training workload portability, and delivered 40% better I/O performance compared to parallel file systems.
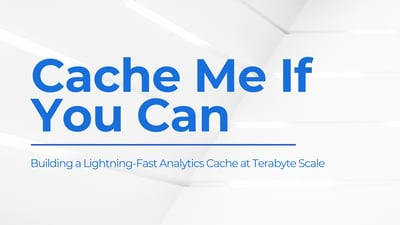
Suresh Kumar Veerapathiran and Anudeep Kumar, engineering leaders at Uptycs, recently shared their experience of evolving their data platform and analytics architecture to power analytics through a generative AI interface. In their post on Medium titled Cache Me If You Can: Building a Lightning-Fast Analytics Cache at Terabyte Scale, Veerapathiran and Kumar provide detailed insights into the challenges they faced (and how they solved them) scaling their analytics solution that collects and reports on terabytes of telemetry data per day as part of Uptycs Cloud-Native Application Protection Platform (CNAPP) solutions.
.png)
.jpeg)
Recently, Qunar deployed Alluxio with Spark in production and found that Alluxio enables Spark streaming jobs to run 15x to 300x faster. In their case study, they described how Alluxio improved their system architecture, and mentioned that some existing Spark jobs would slow down or would never finish because they would run out of memory. After using Alluxio, those jobs were able to finish, because the data could be stored in Alluxio, instead of within Spark. In this blog, we show by saving RDDs in Alluxio, Alluxio can keep larger data sets in-memory for faster Spark applications, as well as enable sharing of RDDs across separate Spark applications.
.jpeg)
Presto was designed from the ground up to offer interactive analytics using a massively parallel processing SQL engine that can combine data from multiple sources using a variety of connectors. As more and more companies discover the power of “separation of storage and compute” along with querying the data where it lies, it’s not wonder Presto is being asked to add even more functionality. Alluxio focuses its innovation at the data layer as a key enabling technology for Presto and a wide range of analytics applications and use cases. Performance is always critical, but providing memory speed response time is only part of the solution. If the application can’t access the data, it’s of no use.
.jpeg)
Caching frequently used data in memory is not a new computing technique, however it is a concept that Alluxio has taken to the next level with the ability to aggregate data from multiple storage systems in a unified pool of memory. Alluxio capabilities extend further to intelligently managing the data within that virtual data layer. Tiered locality uses awareness of network topology and configurable policies to manage data placement for performance and cost optimizations. This feature is particularly useful with cloud deployments across multiple availability zones. It can also be useful for cost savings in environments where cross-zone or cross-location traffic is more expensive than intra-zone data traffic.
.jpeg)
An Alluxio cluster caches data from connected storage systems in memory to create a data layer that can be accessed concurrently by multiple application frameworks. This greatly improves performance for many analytics workloads. On-demand caching occurs when clients read blocks of data using a ‘CACHE’ read type from persistent storage systems connected to the Alluxio cluster. Prior to Alluxio v1.7, on-demand caching was on the critical path of read operations, requiring a full block to be read before the data was available for the application. Workloads which read partial blocks, for example SQL workloads, would be adversely affected on initial reads from connected storage.
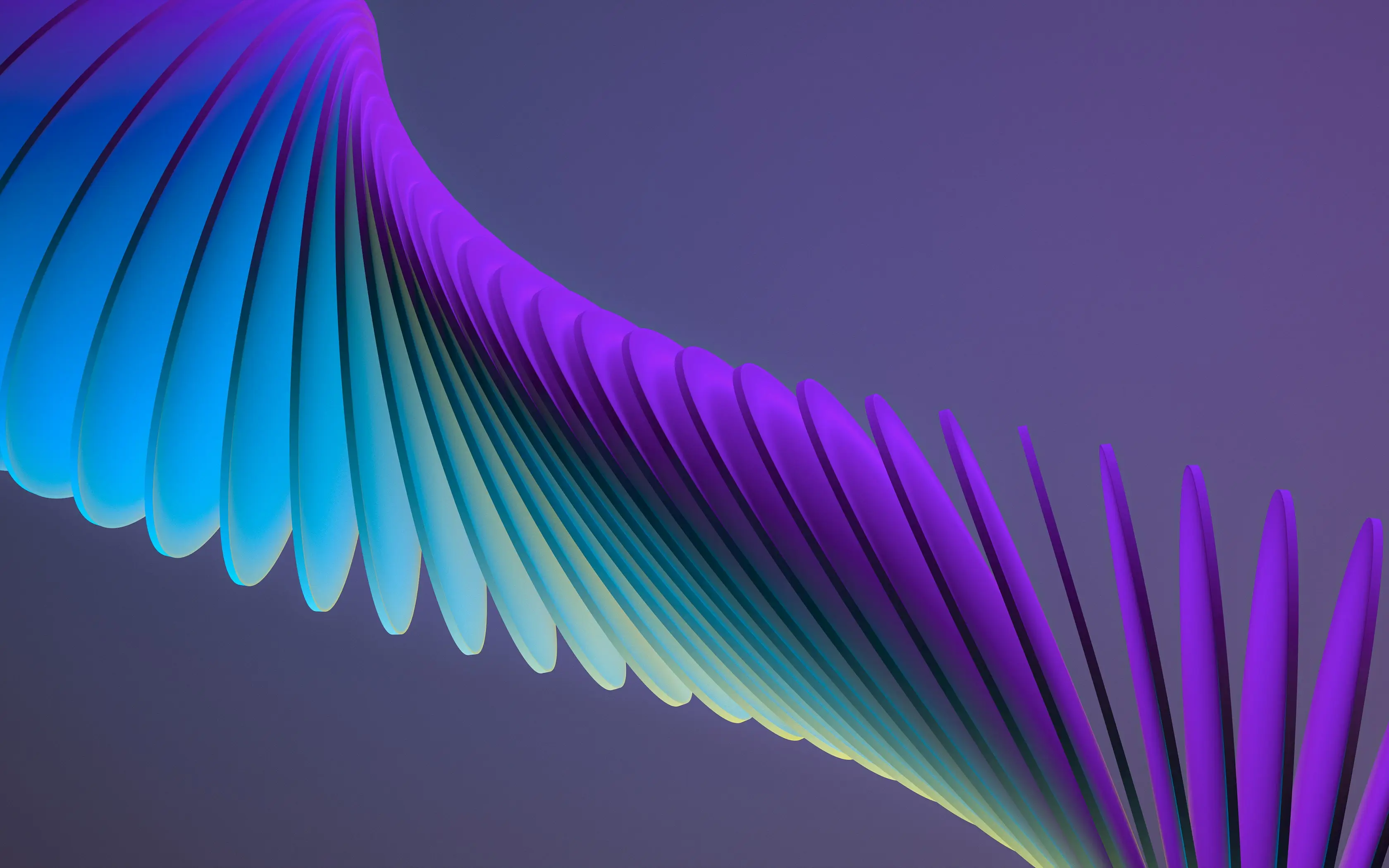
TalkingData leverages Alluxio as a single platform to manage all the data across disparate data sources on-premise and in the cloud. Alluxio removes the complexity of our environment by abstracting the different data sources and providing a unified interface. Applications simply interact with Alluxio, and Alluxio manages data access to different storage systems on behalf of the applications. Alluxio effectively democratizes data access, allowing data scientists and analysts in various business units to accomplish their goals without needing to consider where the data is located or having to go to central IT or the engineering team to transfer or prepare the data.
.jpeg)
While looking for ways to streamline our data pipeline, we learned about Alluxio, an open source, memory speed, virtual distributed file system. We deployed Alluxio as the shared data layer for all of the intermediate stages in the data pipeline. By reading and writing data in Alluxio, the data can be read concurrently and stay in memory for the next stage of the pipeline. This increased the performance by speeding up the entire pipeline, and increased overall throughput of the pipeline allowing us to provide interactive response to our app users.
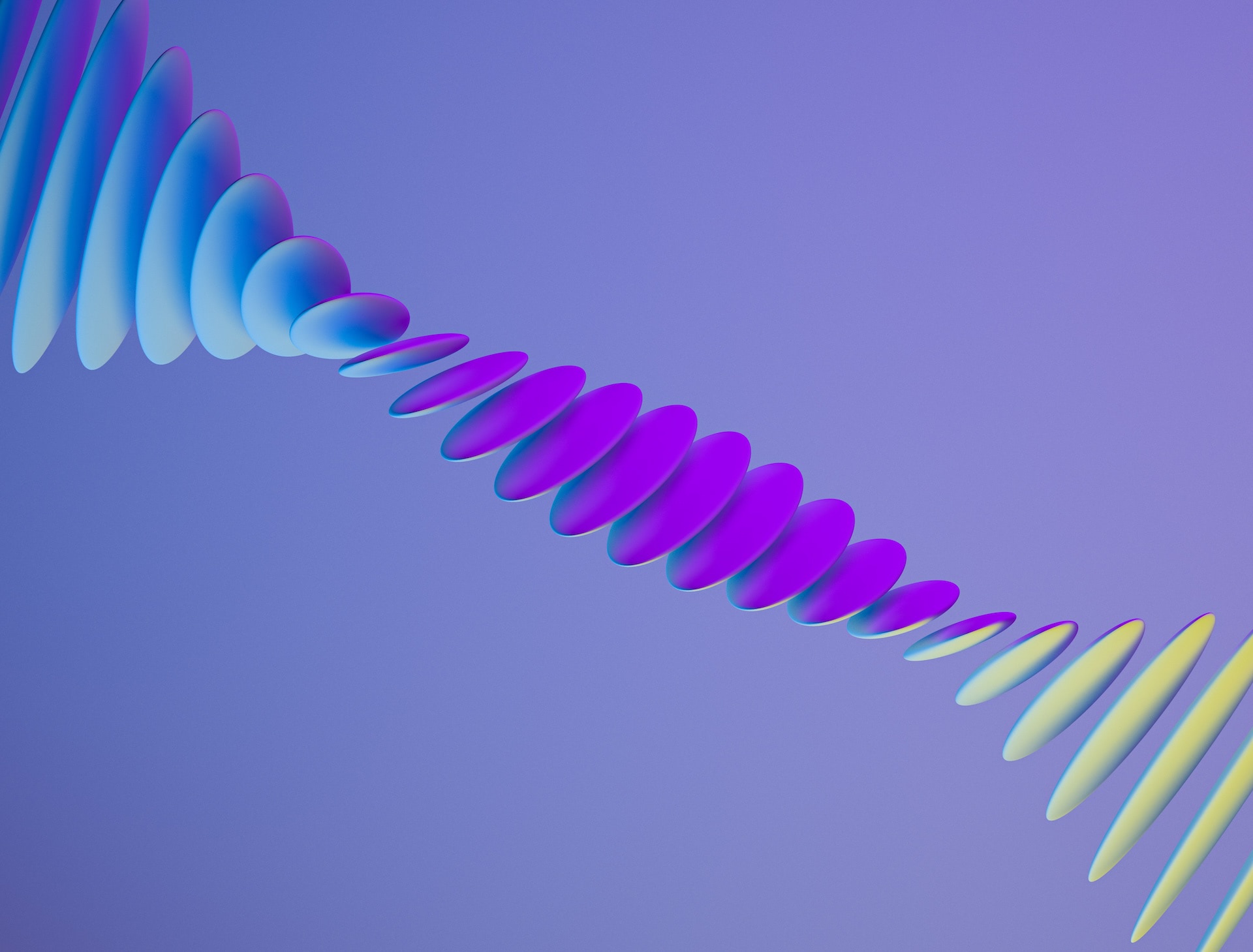
Tencent is one of the largest technology companies in the world and a leader in multiple sectors such as social networking, gaming, e-commerce, mobile and web portal. Tencent News, one of Tencent’s many offerings, strives to create a rich, timely news application to provide users with an efficient, high-quality reading experience. To provide the best experience to more than 100 million monthly active users of Tencent News, we leverage Alluxio with Apache Spark to create a scalable, robust, and performant architecture.
.jpeg)
Alluxio clusters act as a data access accelerator for remote data in connected storage systems. Temporarily storing data in memory, or other media near compute, accelerates access and provides local performance from remote storage. This capability is even more critical with the movement of compute applications to the cloud and data being located in object stores separate from compute. Caching is transparent to users, using read/write buffering to maintain continuity with persistent storage. Intelligent cache management utilizes configurable policies for efficient data placement and supports tiered storage for both memory and disk (SSD/HDD).
.jpeg)
Lenovo is an Alluxio customer with a common problem and use case in the world of data analytics. They have petabytes of data in multiple data centers in different geographic locations. Analyzing it requires an ETL process to get all of the data in the right place. This is both slow, because data has to be transferred across the network, and costly because multiple copies of the data need to be stored. Freshness and quality of the data can also suffer as the data is also potentially out of date and incomplete because regulatory issues prevent certain data from being transferred.
.jpeg)
Alluxio helps organizations handle their big data by providing a unified view of all of the data in your enterprise – on premise, in the cloud, or hybrid. Applications access data using a standard interface to a global virtual namespace. Alluxio also employs a memory-centric architecture to enable data access at memory speed. With the combined unification and performance benefits, Alluxio can effectively provide big data federation for organizations by acting as a virtual data lake.
.jpeg)
The primary appeal of a coupled compute-storage architecture, an architecture where the computation is happening on the machines where the data resides, is the performance possible by bringing the compute engine to the data it requires; however, the costs of maintaining such tight-knit architectures are gradually overtaking the performance benefits. Especially with the popularity of cloud resources, being able to independently scale compute and storage results in large cost savings and cheaper maintenance. In addition, data has become the new oil, and all modern organizations are looking to capture as much data as possible.