This is a recap of the Two Sigma and Alluxio joint meetup hosted in New York. Two Sigma is a leading hedge fund that leverages cutting edge technology to train their models with petabytes of data in on-premise storage. Special thanks to Two Sigma for hosting. Here are the slides from the presentation.
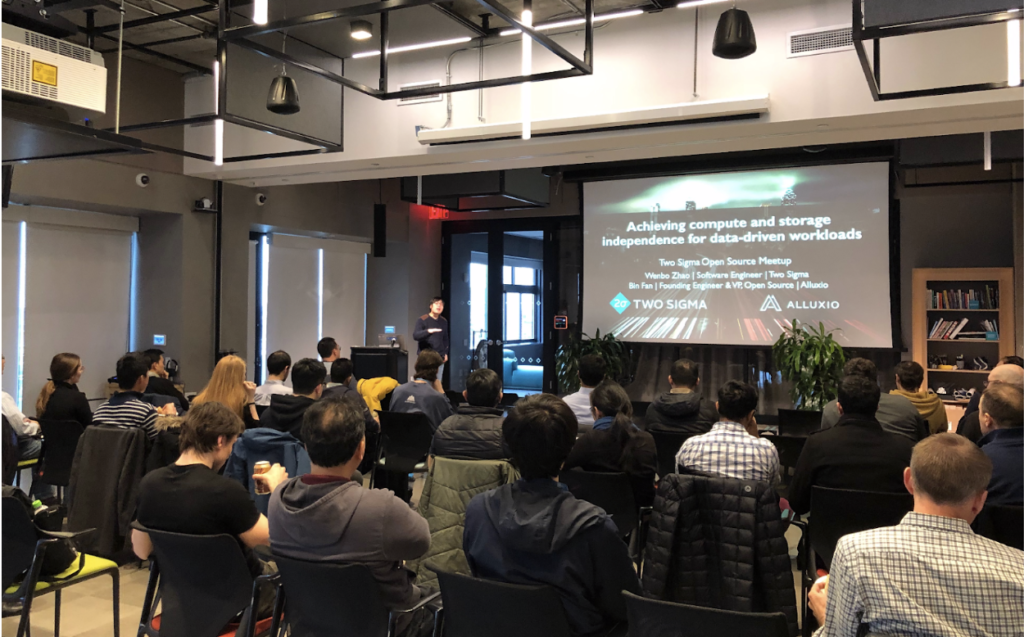
In this meetup, Bin Fan from Alluxio and Wenbo Zhao from Two Sigma co-presented a reference stack (running Alluxio as a data access layer for Apache Spark) that can enable independent and separated compute and storage for big data and machine learning workloads.
Two Sigma’s use case is a great example of the benefits of this reference stack for bursting machine learning computation to the public cloud while still being able to access data stored on-premise efficiently. Their data scientists want to leverage the public cloud as a scalable and elastic computation resource to speed up the end-to-end model training process. By using Alluxio as the data access layer co-located with compute in the cloud, their researchers achieved 10x faster end to end processing, which enables them to perform more iterations on their models.
We had a great time interacting with the audience on the East coast and we look forward to the next NYC event!
To stay up to date on future events, join our meetup groups: Alluxio Open Source New York Meetup, Alluxio Open Source Bay Area Meetup.
If you are interested in hosting or presenting at a future event, please contact us at community@alluxio.com.
.png)
Blog
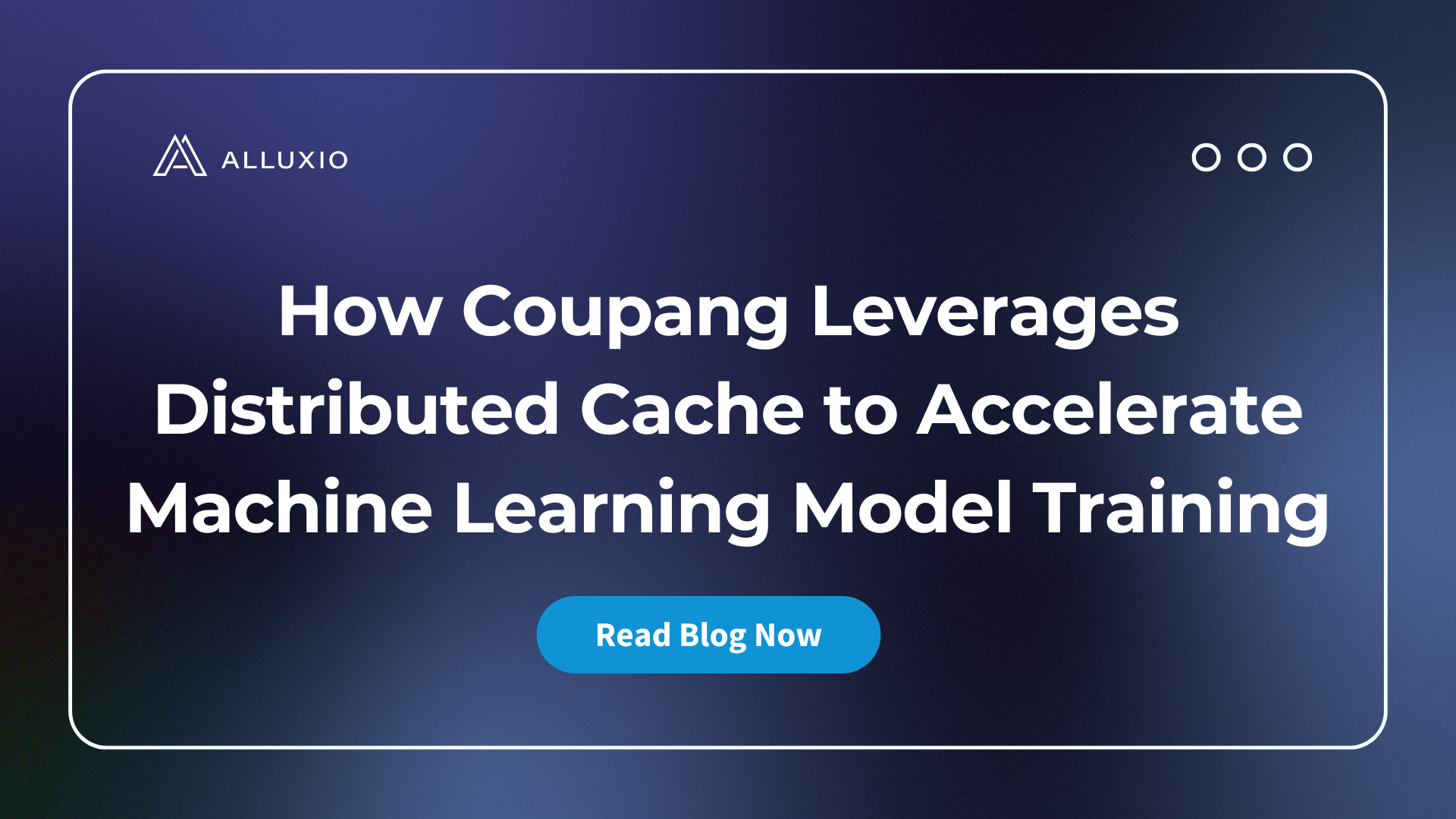
Coupang, a Fortune 200 technology company, manages a multi-cluster GPU architecture for their AI/ML model training. This architecture introduced significant challenges, including:
- Time-consuming data preparation and data copy/movement
- Difficulty utilizing GPU resources efficiently
- High and growing storage costs
- Excessive operational overhead maintaining storage for localized data silos
To resolve these challenges, Coupang’s AI platform team implemented a distributed caching system that automatically retrieves training data from their central data lake, improves data loading performance, unifies access paths for model developers, automates data lifecycle management, and extends easily across Kubernetes environments. The new distributed caching architecture has improved model training speed, reduced storage costs, increased GPU utilization across clusters, lowered operational overhead, enabled training workload portability, and delivered 40% better I/O performance compared to parallel file systems.
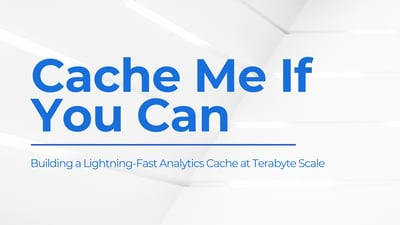
Suresh Kumar Veerapathiran and Anudeep Kumar, engineering leaders at Uptycs, recently shared their experience of evolving their data platform and analytics architecture to power analytics through a generative AI interface. In their post on Medium titled Cache Me If You Can: Building a Lightning-Fast Analytics Cache at Terabyte Scale, Veerapathiran and Kumar provide detailed insights into the challenges they faced (and how they solved them) scaling their analytics solution that collects and reports on terabytes of telemetry data per day as part of Uptycs Cloud-Native Application Protection Platform (CNAPP) solutions.