Blog
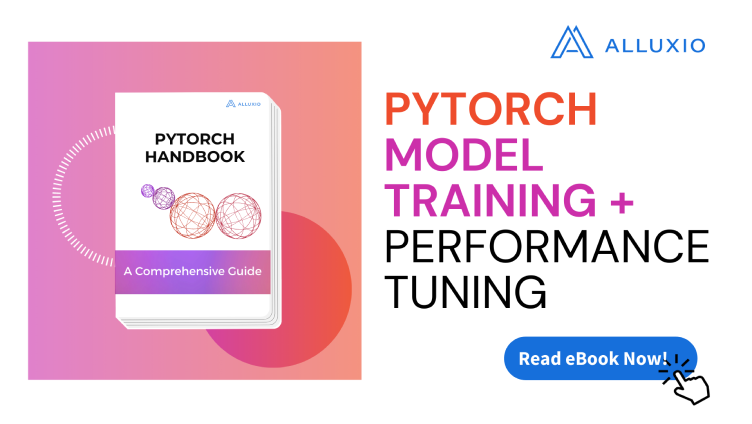
Coupang, a Fortune 200 technology company, manages a multi-cluster GPU architecture for their AI/ML model training. This architecture introduced significant challenges, including:
- Time-consuming data preparation and data copy/movement
- Difficulty utilizing GPU resources efficiently
- High and growing storage costs
- Excessive operational overhead maintaining storage for localized data silos
To resolve these challenges, Coupang’s AI platform team implemented a distributed caching system that automatically retrieves training data from their central data lake, improves data loading performance, unifies access paths for model developers, automates data lifecycle management, and extends easily across Kubernetes environments. The new distributed caching architecture has improved model training speed, reduced storage costs, increased GPU utilization across clusters, lowered operational overhead, enabled training workload portability, and delivered 40% better I/O performance compared to parallel file systems.
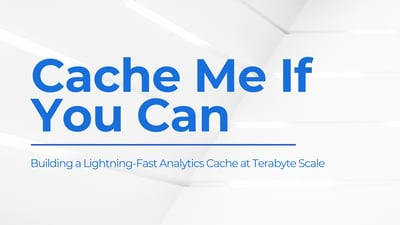
Suresh Kumar Veerapathiran and Anudeep Kumar, engineering leaders at Uptycs, recently shared their experience of evolving their data platform and analytics architecture to power analytics through a generative AI interface. In their post on Medium titled Cache Me If You Can: Building a Lightning-Fast Analytics Cache at Terabyte Scale, Veerapathiran and Kumar provide detailed insights into the challenges they faced (and how they solved them) scaling their analytics solution that collects and reports on terabytes of telemetry data per day as part of Uptycs Cloud-Native Application Protection Platform (CNAPP) solutions.
.png)
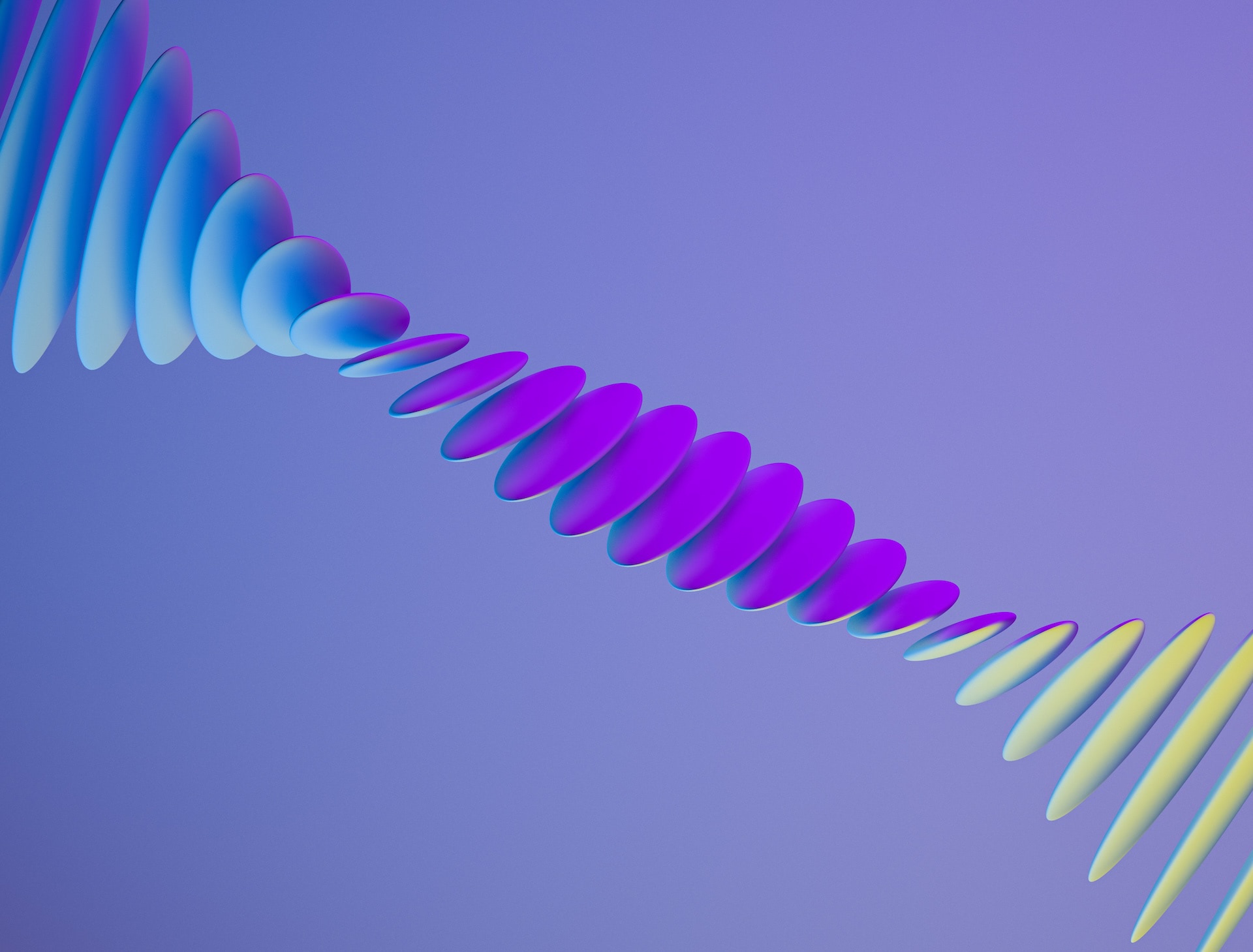


Xi Chen, Senior Software Engineer at Tencent & Top 100 Alluxio open source project contributor, explains the block allocation policy of Alluxio at the code level.
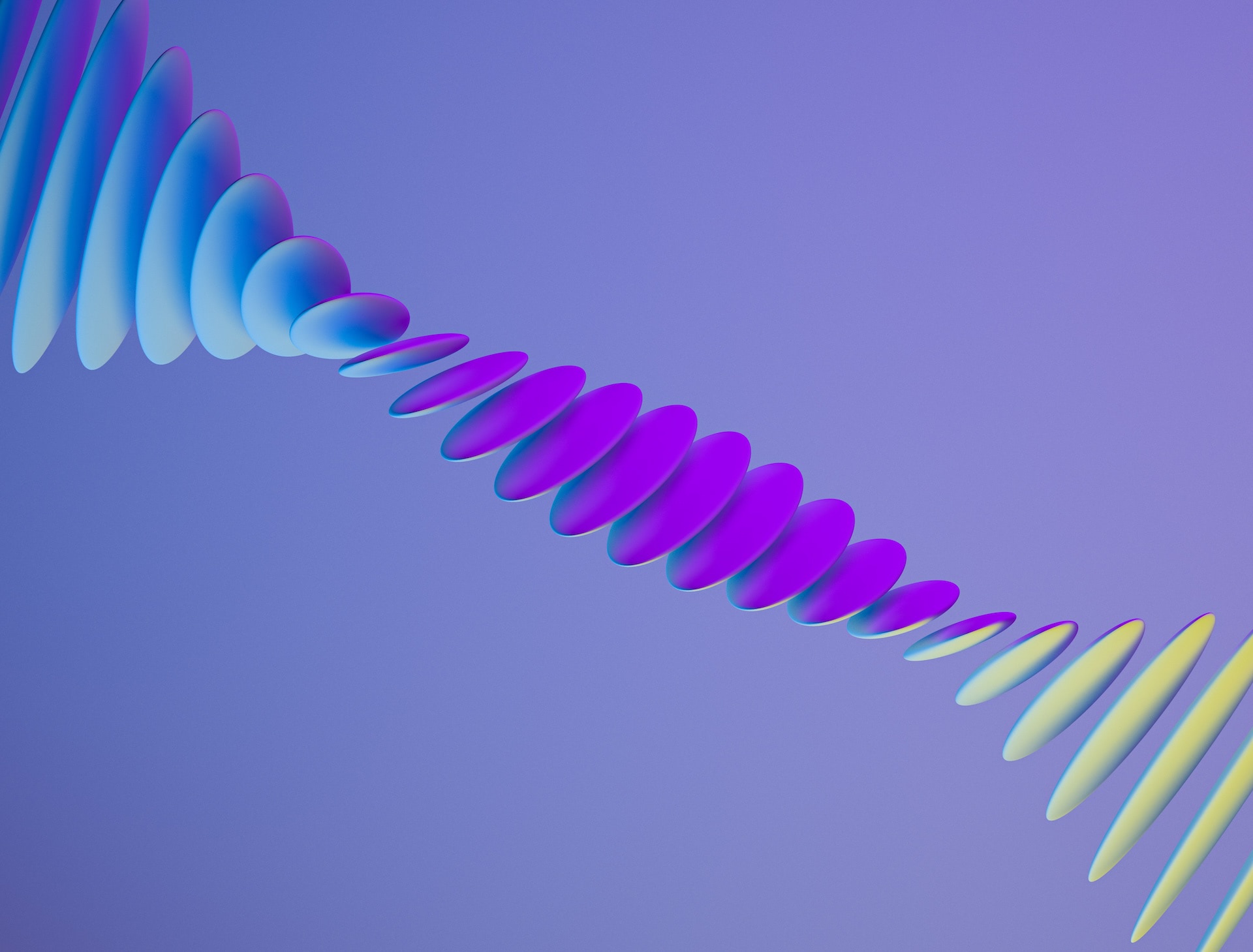
This blog was originally published on the website of NetApp: https://www.netapp.com/blog/modernize-analytics-workloads-netapp-alluxio/
Imagine as an IT leader having the flexibility to choose any services that are available in public cloud and on premises. And imagine being able to scale your storage for your data lakes with control over data locality and protection for your organization. With these goals in mind, NetApp and Alluxio are joining forces to help our customers adapt to new requirements for modernizing data architecture with low-touch operations for analytics, machine learning, and artificial intelligence workflows.
.jpeg)
In the previous blog, we introduced Uber’s Presto use cases and how we collaborated to implement Alluxio local cache to overcome different challenges in accelerating Presto queries. The second part discusses the improvements to the local cache metadata.
.jpeg)
This article shares how Uber and Alluxio collaborated to design and implement Presto local cache to reduce HDFS latency.
.jpeg)
This article introduces the design and implementation of metadata storage in Alluxio Master, either on heap and off heap (based on RocksDB).
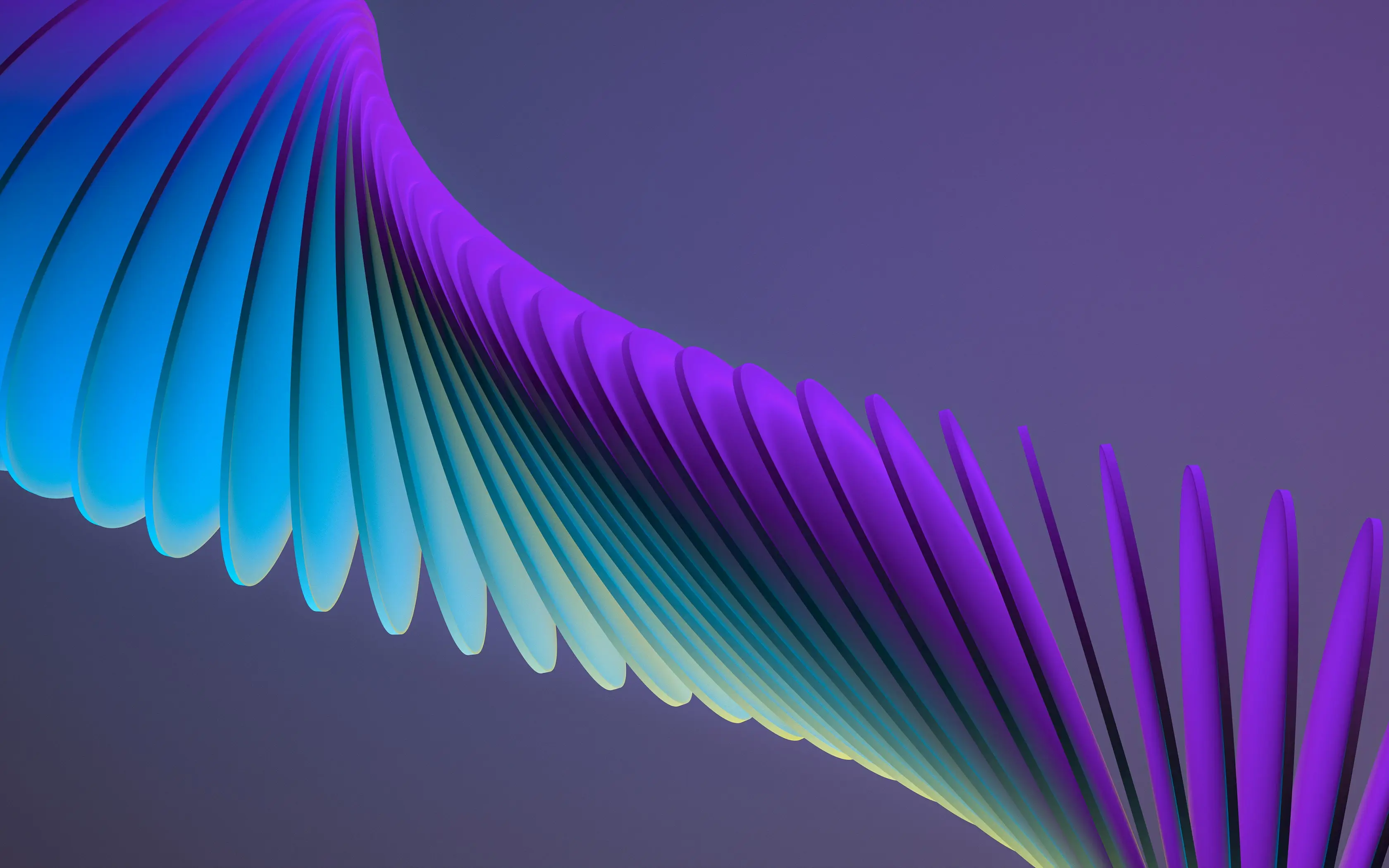
.jpeg)
Raft is an algorithm for state machine replication as a way to ensure high availability (HA) and fault tolerance. This blog shares how Alluxio has moved to a Zookeeper-less, built-in Raft-based journal system as a HA implementation.
.jpeg)
With machine learning (ML) and artificial intelligence (AI) applications becoming more business-critical, organizations are in the race to advance their AI/ML capabilities. To realize the full potential of AI/ML, having the right underlying machine learning platform is a prerequisite.
.jpeg)
This article will discuss a new solution to orchestrating data for end-to-end machine learning pipelines that addresses the above questions. I will outline common challenges and pitfalls, followed by proposing a new technique, data orchestration, to optimize the data pipeline for machine learning.